Motor imagery electroencephalogram voting strategy sorting method based on extreme learning machines
An extremely fast learning machine and motor imagery technology, applied in the field of pattern recognition and brain-computer interface, can solve the problem of not reducing the randomness of sample prediction categories, and achieve the effects of improving classification accuracy, reducing randomness, and low time consumption
- Summary
- Abstract
- Description
- Claims
- Application Information
AI Technical Summary
Problems solved by technology
Method used
Image
Examples
Embodiment Construction
[0019] The present invention will be further described below in combination with specific embodiments.
[0020] Suppose there is a training data set TrainData and a set of test data sets TestData, the sample size of TrainData is N, and the dimension is D; the sample size of TestData is M, and the dimension is also D. Among them, the samples in TrainData and TestData belong to K categories.
[0021] Voting strategy classification method for motor imagery EEG signals based on extremely fast learning machine, the flow chart is as follows figure 2 shown.
[0022] Step 1: Divide the TrainData and TestData into S-segment EEG signals by means of fixed time window division. TrainData i Represents the i-th sub-signal in the training data set, and the dimension of each sub-signal is D i (i=1,2,...,S). TestData i Represents the i-th sub-signal in the test data set, and the dimension of each sub-signal is D i (i=1,2,...,S). Because a fixed time window is used, the window size is ...
PUM
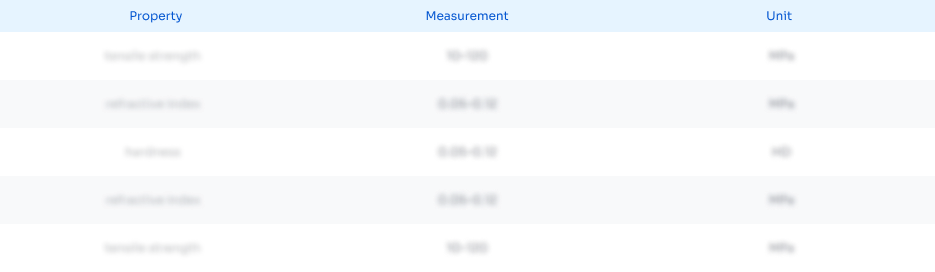
Abstract
Description
Claims
Application Information
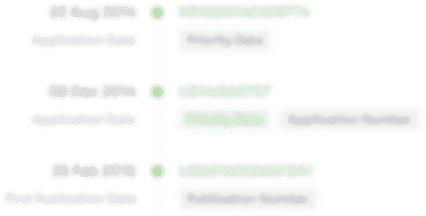
- R&D Engineer
- R&D Manager
- IP Professional
- Industry Leading Data Capabilities
- Powerful AI technology
- Patent DNA Extraction
Browse by: Latest US Patents, China's latest patents, Technical Efficacy Thesaurus, Application Domain, Technology Topic, Popular Technical Reports.
© 2024 PatSnap. All rights reserved.Legal|Privacy policy|Modern Slavery Act Transparency Statement|Sitemap|About US| Contact US: help@patsnap.com