Self-adaptive high-order volume Kalman filtering method
A Kalman filter and high-order volume technology, applied in the field of signal processing, can solve problems such as filter performance degradation and system state mutation, and achieve the effects of suppressing filter errors and strong tracking capabilities
- Summary
- Abstract
- Description
- Claims
- Application Information
AI Technical Summary
Problems solved by technology
Method used
Image
Examples
Embodiment Construction
[0018] The present invention will be further described below in conjunction with the accompanying drawings and embodiments.
[0019] refer to figure 1 , let the state-space model of the nonlinear dynamic system be:
[0020] x(k+1)=f(x(k))+w(k)
[0021] z(k)=h(x(k))+v(k)
[0022] where x(k)∈R n Indicates the system state (R n is the complete set of n-dimensional column vectors), z(k)∈R m is the measurement vector, f(x(k)) and h(x(k)) are differentiable functions, w(k)∈R n and v(k)∈R m Both are Gaussian white noise with zero mean, and their variances are Q(k) and R(k) respectively, and the system noise variance Q(k) is time-varying unknown.
[0023] Suppose the initial state of the system is: P(0|0)=p(0), and x(0) is independent of w(k) and v(k), respectively.
[0024] Below, based on the system model, the specific implementation steps of AHCKF are described in detail:
[0025] Step 1 sets the initial conditions for filtering: P(0|0)=p(0),
[0026] Step 2 time up...
PUM
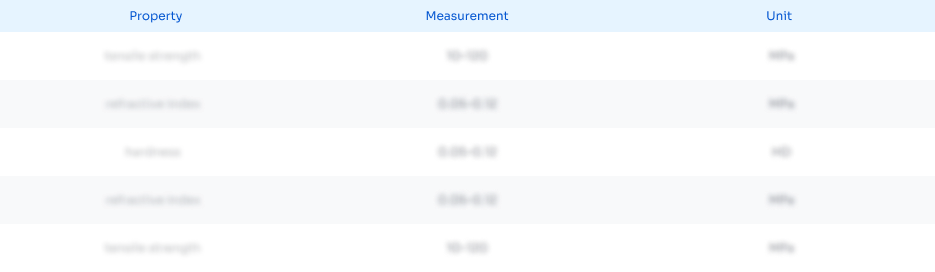
Abstract
Description
Claims
Application Information
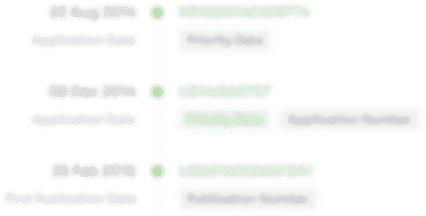
- R&D Engineer
- R&D Manager
- IP Professional
- Industry Leading Data Capabilities
- Powerful AI technology
- Patent DNA Extraction
Browse by: Latest US Patents, China's latest patents, Technical Efficacy Thesaurus, Application Domain, Technology Topic, Popular Technical Reports.
© 2024 PatSnap. All rights reserved.Legal|Privacy policy|Modern Slavery Act Transparency Statement|Sitemap|About US| Contact US: help@patsnap.com