Residential load prediction method of elman-based neural network
A neural network and load forecasting technology, applied in forecasting, instrumentation, data processing applications, etc., can solve problems such as low accuracy and scattered distribution
- Summary
- Abstract
- Description
- Claims
- Application Information
AI Technical Summary
Problems solved by technology
Method used
Image
Examples
Embodiment Construction
[0039] For the convenience of the following description, the following noun explanations are first given:
[0040] Elman network: J.L.Elman first proposed it for speech processing in 1990. It is a typical local regression network (global feed for ward local recurrent).
[0041] BP algorithm: Error Back Propagation Algorithm, error back propagation algorithm, referred to as BP algorithm.
[0042] refer to figure 1 , the present invention provides a kind of resident load forecasting method based on elman neural network, comprising:
[0043] S1. Obtain the historical data of residents' load and the corresponding historical weather parameter data in the previous year, and at the same time divide the valid days in the year into date types;
[0044] S2. According to the acquired historical data of resident load, calculate the average number of residents' load in each month, and then calculate the total average value of all averages in the same period, and divide each average numbe...
PUM
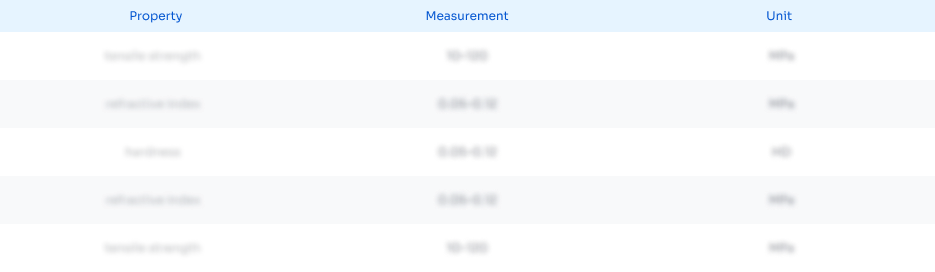
Abstract
Description
Claims
Application Information
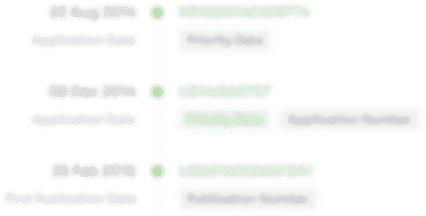
- R&D Engineer
- R&D Manager
- IP Professional
- Industry Leading Data Capabilities
- Powerful AI technology
- Patent DNA Extraction
Browse by: Latest US Patents, China's latest patents, Technical Efficacy Thesaurus, Application Domain, Technology Topic, Popular Technical Reports.
© 2024 PatSnap. All rights reserved.Legal|Privacy policy|Modern Slavery Act Transparency Statement|Sitemap|About US| Contact US: help@patsnap.com