Fabric defect detection method based on sparse representation coefficient optimization
A technique of sparsely representing coefficients and sparse coefficients, applied in image data processing, instruments, calculations, etc., can solve the problems of large reconstruction errors, false detections, and missed detections of original fabrics
- Summary
- Abstract
- Description
- Claims
- Application Information
AI Technical Summary
Problems solved by technology
Method used
Image
Examples
Embodiment
[0087] In the embodiment, images of common defects in the fabric image library are used for experiments, including images of leaking yarn, damage, loose weft, jumping flowers, knots, etc. The size of the image is 256×256, select some images such as figure 2 (a)-(f) in. In the embodiment, the value of k is 4, the value of λ in the formula (1) is 0.05, and the value of γ in the formula (3) is 1.5. Using the sparse representation coefficient matrix α, α before and after optimization * and the dictionary library D to reconstruct the fabric image to be tested, the results are as follows image 3 (a)-(f) and Figure 4 (a)-(f) in. Depend on image 3 (a)-(f) and Figure 4 (a)-(f) are compared one by one, and the image reconstructed by using the sparse representation coefficient matrix α is image 3 In (a)-(f) there are relatively obvious defect areas, and the optimized sparse representation coefficient matrix α is used * owned Figure 4 The reconstructed images in (a)-(f) ar...
PUM
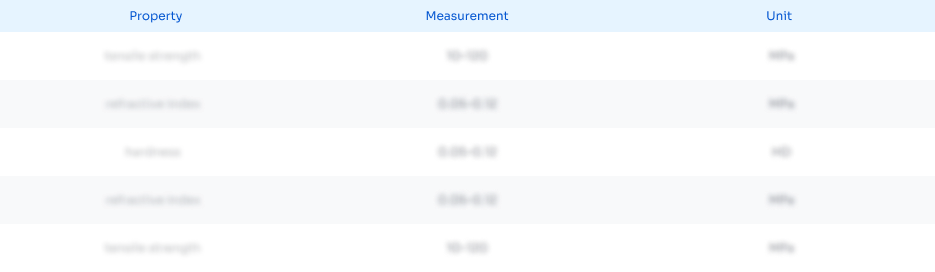
Abstract
Description
Claims
Application Information
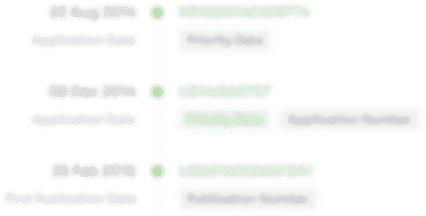
- R&D Engineer
- R&D Manager
- IP Professional
- Industry Leading Data Capabilities
- Powerful AI technology
- Patent DNA Extraction
Browse by: Latest US Patents, China's latest patents, Technical Efficacy Thesaurus, Application Domain, Technology Topic, Popular Technical Reports.
© 2024 PatSnap. All rights reserved.Legal|Privacy policy|Modern Slavery Act Transparency Statement|Sitemap|About US| Contact US: help@patsnap.com