Rolling bearing fault diagnosis method based on SIFT-KPCA and SVM
A fault diagnosis, rolling bearing technology, applied in the direction of mechanical bearing testing, measuring devices, instruments, etc., to achieve strong robustness, reduce the amount of calculation, and improve the speed of operation
- Summary
- Abstract
- Description
- Claims
- Application Information
AI Technical Summary
Problems solved by technology
Method used
Image
Examples
Embodiment Construction
[0028] The present invention will be further described below in conjunction with the accompanying drawings and specific embodiments.
[0029] Such as figure 1 Shown is the overall framework of a rolling bearing fault diagnosis method based on SIFT-KPCA and SVM in the present invention. Before applying the image processing algorithm, the vibration signal is first converted into a two-dimensional image, and wavelet noise reduction is used before the conversion to reduce the interference of noise on feature extraction. Then, the SIFT algorithm is applied to the two-dimensional image to extract the scale-invariant feature vector, and a 128-dimensional feature matrix is obtained, and then the KPCA algorithm is used to realize the dimensionality reduction of the feature vector. Thereafter, the singular values of the simplified eigenvectors are extracted and finally input into the SVM classifier to realize fault classification. The fault diagnosis method in the present inventio...
PUM
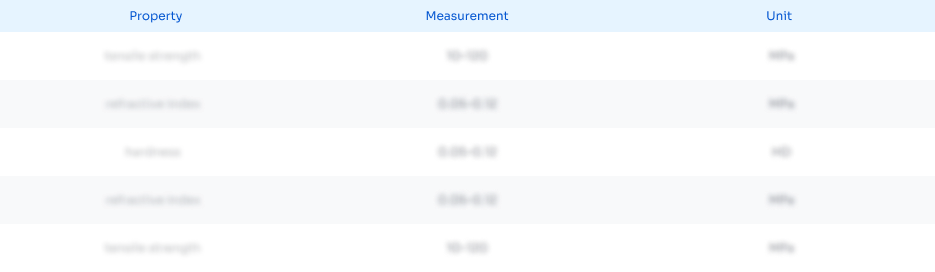
Abstract
Description
Claims
Application Information
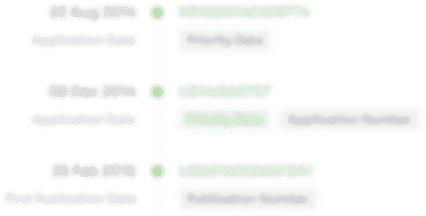
- R&D Engineer
- R&D Manager
- IP Professional
- Industry Leading Data Capabilities
- Powerful AI technology
- Patent DNA Extraction
Browse by: Latest US Patents, China's latest patents, Technical Efficacy Thesaurus, Application Domain, Technology Topic.
© 2024 PatSnap. All rights reserved.Legal|Privacy policy|Modern Slavery Act Transparency Statement|Sitemap