Method for constructing and training dynamic neural network of incomplete recursive support
A dynamic neural network and neural network technology, applied in the field of incomplete recursive support dynamic neural network and its learning algorithms, can solve problems such as time-consuming training, time-consuming and labor-intensive, and inability to fundamentally overcome the BP algorithm.
- Summary
- Abstract
- Description
- Claims
- Application Information
AI Technical Summary
Problems solved by technology
Method used
Image
Examples
Embodiment Construction
[0038] In essence, neural networks can be divided into two categories: feed-forward networks without feedback and recurrent networks with feedback. Because there is no feedback, the multi-layer forward network has a simple structure and a mature learning algorithm; but it is essentially a static structure, which cannot describe the dynamic system well, which limits its use in neural network control. The feedback characteristics of the recurrent network make it have a good ability to express the dynamic system, such as the attractor dynamic structure, historical information storage and other characteristics, so that it has obtained great application advantages in the dynamic system. Unfortunately, due to the complex structure and dynamics of the fully connected dynamic recurrent neural network, there are too many bidirectional weight parameters, and the feedback recursion makes the learning process complicated and lengthy, which in turn restricts its wide application. Based on ...
PUM
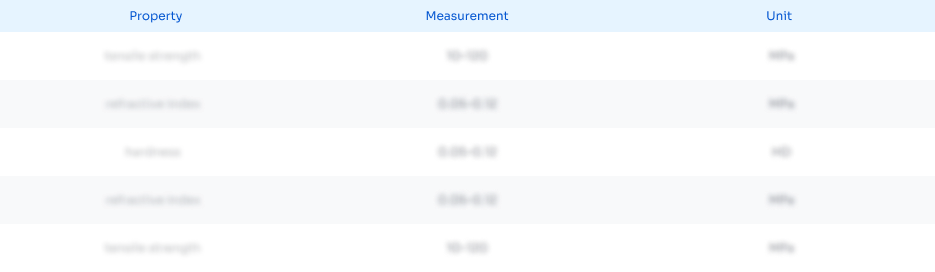
Abstract
Description
Claims
Application Information
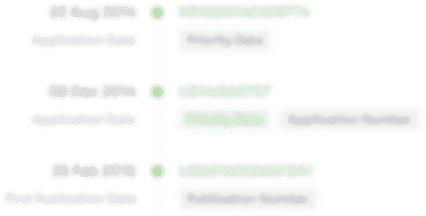
- R&D Engineer
- R&D Manager
- IP Professional
- Industry Leading Data Capabilities
- Powerful AI technology
- Patent DNA Extraction
Browse by: Latest US Patents, China's latest patents, Technical Efficacy Thesaurus, Application Domain, Technology Topic, Popular Technical Reports.
© 2024 PatSnap. All rights reserved.Legal|Privacy policy|Modern Slavery Act Transparency Statement|Sitemap|About US| Contact US: help@patsnap.com