Empirical mode neural network-based chaotic time series prediction method
A technology of chaotic time series and empirical mode, applied in biological neural network model, prediction, data processing application, etc., can solve the problems of adaptive limitation of wavelet neural network, difficulty in determining the number of hidden layers of neural network, etc. The effect of improving self-adaptation, enhancing self-adaptation, strong adaptability and robustness
- Summary
- Abstract
- Description
- Claims
- Application Information
AI Technical Summary
Problems solved by technology
Method used
Image
Examples
Embodiment Construction
[0031] like figure 1 As shown, the chaotic time series prediction method based on empirical modal neural network includes the following steps:
[0032] 1) Discrimination of chaotic characteristics of chaotic time series. The basic characteristic of chaotic motion is that motion is extremely sensitive to initial conditions. The orbits generated by two very close initial values separate exponentially over time, and the Lyapunov exponent is the quantity that describes this phenomenon. Gribo proved that as long as the maximum Lyapunov exponent of the sequence is greater than zero, the existence of chaos can be confirmed. Since the Wolf method is suitable for time series without noise, the evolution of small vectors in the tangent space is highly nonlinear. In this implementation example, the Wolf method is selected to obtain the sequence The largest Lyapunov exponent of , and use it to distinguish nonlinear time series Whether there is chaos.
[0033] 2) Chaotic time seri...
PUM
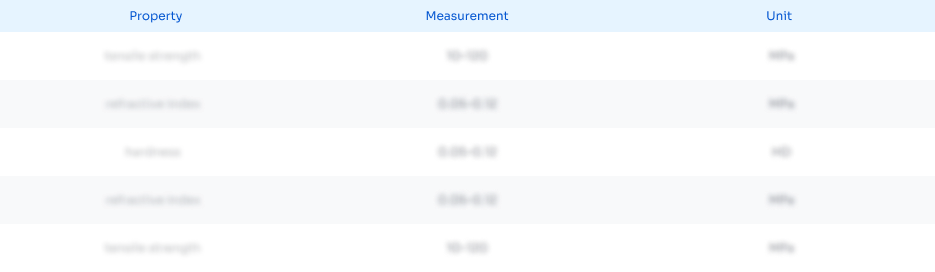
Abstract
Description
Claims
Application Information
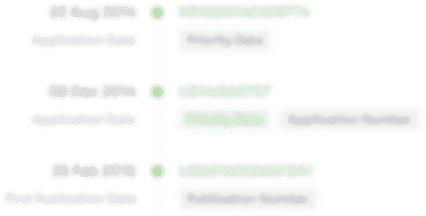
- Generate Ideas
- Intellectual Property
- Life Sciences
- Materials
- Tech Scout
- Unparalleled Data Quality
- Higher Quality Content
- 60% Fewer Hallucinations
Browse by: Latest US Patents, China's latest patents, Technical Efficacy Thesaurus, Application Domain, Technology Topic, Popular Technical Reports.
© 2025 PatSnap. All rights reserved.Legal|Privacy policy|Modern Slavery Act Transparency Statement|Sitemap|About US| Contact US: help@patsnap.com