Nucleation classifier based on local spline embedding
A local spline embedding and classifier technology, which is applied in the direction of instruments, computer components, character and pattern recognition, etc., to achieve the effect of facilitating processing and improving accuracy
- Summary
- Abstract
- Description
- Claims
- Application Information
AI Technical Summary
Problems solved by technology
Method used
Image
Examples
Embodiment Construction
[0024] Main technical idea of the present invention is:
[0025] The present invention adopts the non-linear dimension reduction algorithm based on local spline embedding plus the linear SVM (linear support vector machine) classification algorithm to carry out dimension reduction classification to high-dimensional labeled data, and integrates supervisory information to overcome the local spline embedding algorithm The result of high-dimensional information dimensionality reduction is not necessarily conducive to the defects of classification processing. At the same time, constructing intra-class graphs and inter-class graphs to distinguish intra-class neighbors from inter-class neighbors is extremely effective in achieving intra-class compactness and inter-class discreteness. Great help, especially the introduction of regenerating kernel Hilbert spaces, using kernel methods to find the best nonlinear embedding of test data, and being able to handle classification problems tha...
PUM
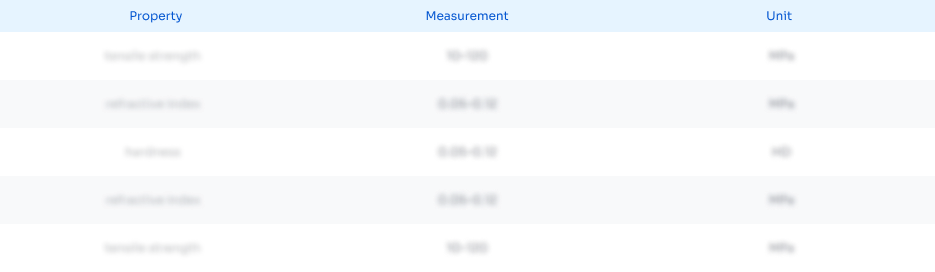
Abstract
Description
Claims
Application Information
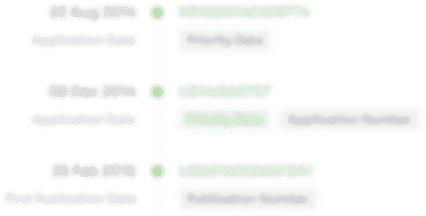
- R&D
- Intellectual Property
- Life Sciences
- Materials
- Tech Scout
- Unparalleled Data Quality
- Higher Quality Content
- 60% Fewer Hallucinations
Browse by: Latest US Patents, China's latest patents, Technical Efficacy Thesaurus, Application Domain, Technology Topic, Popular Technical Reports.
© 2025 PatSnap. All rights reserved.Legal|Privacy policy|Modern Slavery Act Transparency Statement|Sitemap|About US| Contact US: help@patsnap.com