Deep learning-based quick dynamic human body action extraction and identification method
A human motion and deep learning technology, applied in the field of motion recognition, can solve the problems of large hardware occupation and reduce hardware requirements, and achieve the effect of reducing hardware requirements and solving excessive hardware occupation.
- Summary
- Abstract
- Description
- Claims
- Application Information
AI Technical Summary
Problems solved by technology
Method used
Image
Examples
Embodiment 1
[0016] A fast dynamic human action extraction and recognition method based on deep learning. First, it describes the overall information of the size, color, edge, outline, shape and depth of the human target, provides useful clues for action recognition, and extracts effective information from video sequences. In the long-range case, the target’s trajectory is used for trajectory analysis; in the close-up case, it is necessary to use the information extracted from the image sequence to model the target’s limbs and torso in 2D or 3D.
Embodiment 2
[0018] According to the deep learning-based fast dynamic human body action extraction and recognition method described in Embodiment 1, by searching for features such as human body size, color, edge, outline, shape, etc., it is determined that the moving object is a human being, and then the human body image is intercepted by screening, and then in Mark points that can be identified and tracked are set on the main joint positions or more positions on the human body, and the movement of the same human body is captured by the camera, and then according to the spatial geometric parameters, combined with some digital models of human motion, it is possible to calculate the The position of each mark point at each moment, the combination of multiple mark point positions constitutes the overall position of the human body, and continuous position recognition is performed to identify human body movements.
Embodiment 3
[0020] According to the method for extracting and recognizing fast dynamic human body movements based on deep learning described in embodiment 1 or 2, the image collected by the camera of the video camera is processed in real time, first collects and distinguishes the people in the image, and frames the area where pedestrians are located Select it, and then compare each frame of the area with its previous frame and the next frame to calculate the movement changes of pixels in the three frames. By calculating the OpticalFlow of pixel movement, the displacement vector of pixel movement (Fx , Fy), and then decompose the vector so that: After being filtered by a Gaussian filter, the feature representation of the pedestrian's action of interest is obtained;
[0021] Motion feature image see attached figure 2
[0022] Feature calculation formula:
[0023] .
PUM
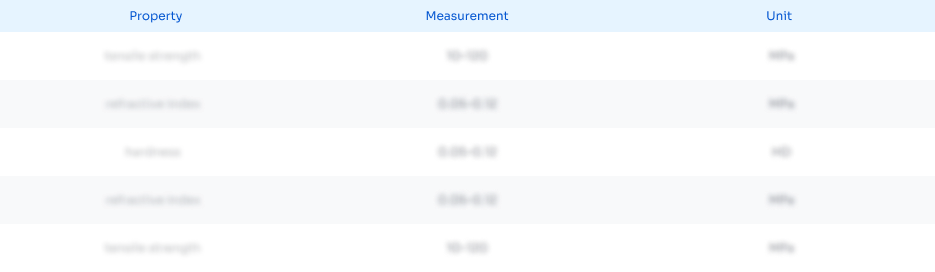
Abstract
Description
Claims
Application Information
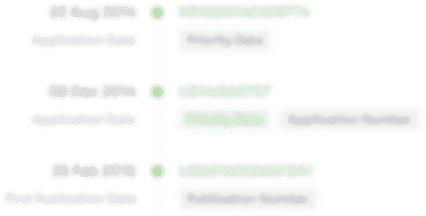
- R&D Engineer
- R&D Manager
- IP Professional
- Industry Leading Data Capabilities
- Powerful AI technology
- Patent DNA Extraction
Browse by: Latest US Patents, China's latest patents, Technical Efficacy Thesaurus, Application Domain, Technology Topic, Popular Technical Reports.
© 2024 PatSnap. All rights reserved.Legal|Privacy policy|Modern Slavery Act Transparency Statement|Sitemap|About US| Contact US: help@patsnap.com