Hyperspectral image classification based on gradient lifting decision tree and semi-supervise algorithm integration
A hyperspectral image and decision tree technology, applied in computing, computer parts, instruments, etc., can solve problems such as the decline of classifier learning performance, the need to improve the classification accuracy, and the inaccurate prediction of class labels, so as to ensure the accuracy of classification, The effect of reducing the number and improving the accuracy
- Summary
- Abstract
- Description
- Claims
- Application Information
AI Technical Summary
Problems solved by technology
Method used
Image
Examples
Embodiment Construction
[0028] The present invention will be further described below in conjunction with the accompanying drawings and embodiments.
[0029] refer to figure 1 , the specific implementation steps of the present invention include:
[0030] Step 1, input hyperspectral image data:
[0031] Input a hyperspectral image, remove the background sample points, and there are N remaining sample points, including C categories.
[0032] Step 2, sample point spatial spectrum feature extraction, the implementation steps are:
[0033] In step 2a, the spectral feature values of each band of each sample point are used as the spectral feature vector of the sample point, and the original feature dimension of the sample point is d.
[0034] Step 2b, take the neighborhood window for each sample point, the window size is c×c, take the maximum value of each dimension feature of all sample points in the window as the spatial feature of the central sample point, and the feature dimension is d.
[0035] In...
PUM
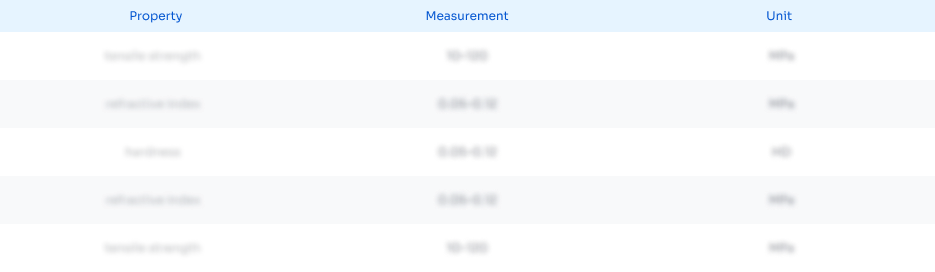
Abstract
Description
Claims
Application Information
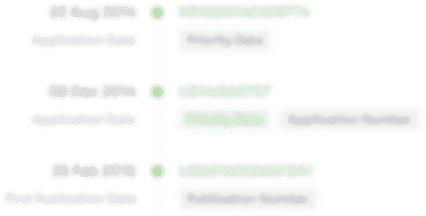
- R&D Engineer
- R&D Manager
- IP Professional
- Industry Leading Data Capabilities
- Powerful AI technology
- Patent DNA Extraction
Browse by: Latest US Patents, China's latest patents, Technical Efficacy Thesaurus, Application Domain, Technology Topic, Popular Technical Reports.
© 2024 PatSnap. All rights reserved.Legal|Privacy policy|Modern Slavery Act Transparency Statement|Sitemap|About US| Contact US: help@patsnap.com