Multiple dimensioned convolution neural network-based real time human body abnormal behavior identification method
A convolutional neural network and recognition method technology, applied in the field of detection of abnormal human behavior in video, can solve problems such as false positives and false negatives, missing extraction areas, and poor recognition, etc., to improve flexibility and Application range, high recognition rate, satisfying the effect of real-time monitoring
- Summary
- Abstract
- Description
- Claims
- Application Information
AI Technical Summary
Problems solved by technology
Method used
Image
Examples
Embodiment Construction
[0050] In order to facilitate those skilled in the art to understand the technical content of the present invention, the content of the present invention will be further explained below in conjunction with the accompanying drawings.
[0051] The technical solution of the present invention is: a real-time abnormal human behavior recognition method based on a multi-scale convolutional neural network, including:
[0052] S1. Determine the structure of the multi-scale convolutional neural network; such as figure 1 As shown, it includes the first layer, the second layer, the third layer, the fourth layer, the fifth layer, the sixth layer and the seventh layer; each layer contains several nodes, and the number of nodes determines the number of extracted features. The more data there is, the more feature information is extracted, but the amount of calculation is also greater.
[0053] The first layer is the input layer input, which contains three channels, which respectively accept ...
PUM
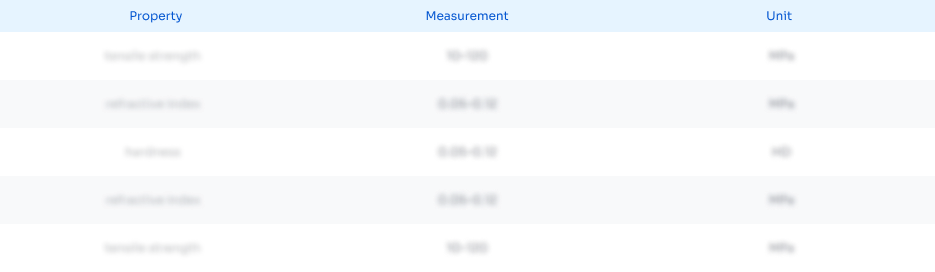
Abstract
Description
Claims
Application Information
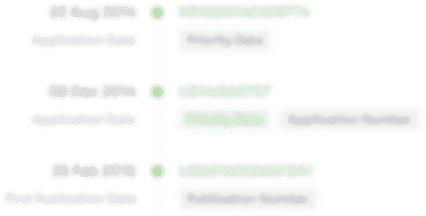
- R&D Engineer
- R&D Manager
- IP Professional
- Industry Leading Data Capabilities
- Powerful AI technology
- Patent DNA Extraction
Browse by: Latest US Patents, China's latest patents, Technical Efficacy Thesaurus, Application Domain, Technology Topic.
© 2024 PatSnap. All rights reserved.Legal|Privacy policy|Modern Slavery Act Transparency Statement|Sitemap