Data classification method based on self-set density search and clustering detector-negative selection algorithm (DSC-NSA)
A technology of data classification and negative selection, applied in computing, computer parts, instruments, etc., can solve the problems of small time overhead, low false positive rate, high false positive rate, and achieve the goal of small time cost, elimination of influence, and elimination of noise. Effect
- Summary
- Abstract
- Description
- Claims
- Application Information
AI Technical Summary
Problems solved by technology
Method used
Image
Examples
Embodiment Construction
[0047] The present invention will be further described below in conjunction with the accompanying drawings.
[0048] refer to Figure 1 ~ Figure 4 , a data classification method based on the negative selection algorithm of self-set density search and partition clustering, the data classification method includes the following steps:
[0049] 1) Calculate the cluster center and delete the noise according to the density peak value of the data set;
[0050] 2) Generate a self-detector from non-noisy self-samples;
[0051] 3) Generate non-self detectors from self detectors;
[0052] 4) Use the self-detector and the non-self-detector at the same time to judge whether the detected data samples are abnormal, and realize data classification.
[0053] refer to figure 1 and figure 2 , the self-set clustering process is as follows:
[0054] 1.1) Calculate the ρ of each point in the data set according to the formula i and δ i , ρ i is the density of the i-th data point, δ i is t...
PUM
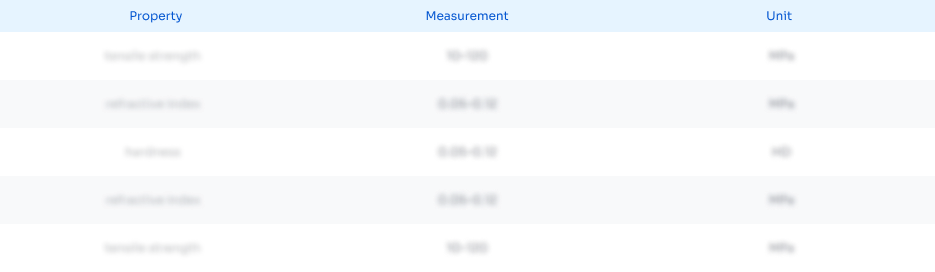
Abstract
Description
Claims
Application Information
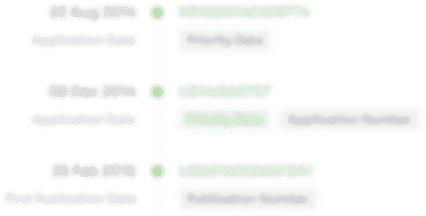
- R&D Engineer
- R&D Manager
- IP Professional
- Industry Leading Data Capabilities
- Powerful AI technology
- Patent DNA Extraction
Browse by: Latest US Patents, China's latest patents, Technical Efficacy Thesaurus, Application Domain, Technology Topic, Popular Technical Reports.
© 2024 PatSnap. All rights reserved.Legal|Privacy policy|Modern Slavery Act Transparency Statement|Sitemap|About US| Contact US: help@patsnap.com