Motion imagery electroencephalograph classification method based on multilayer extreme learning machine
An ultra-limited learning machine and EEG technology, which is applied in the field of motor imagery EEG signal classification to achieve the effect of low time consumption and improved classification accuracy.
- Summary
- Abstract
- Description
- Claims
- Application Information
AI Technical Summary
Problems solved by technology
Method used
Image
Examples
Embodiment Construction
[0017] The present invention will be further described below in conjunction with the accompanying drawings and specific embodiments.
[0018] Suppose there is a training data set TrainData and a set of test data sets TestData, the sample size of TrainData is N, and the dimension is D; the sample size of TestData is M, and the dimension is also D. Among them, the samples in TrainData and TestData belong to K categories.
[0019] Motor imagery EEG signal classification method based on multi-layer extreme learning machine, the flow chart is as follows figure 1 shown.
[0020] Step 1: Divide the TrainData and TestData into S-segment EEG signals by means of fixed time window division. TrainData i Represents the i-th sub-signal in the training data set, and the dimension of each sub-signal is D i (i=1,2,...,S). TestData i Represents the i-th sub-signal in the test data set, and the dimension of each sub-signal is D i (i=1,2,...,S). Because of the fixed time window used, the ...
PUM
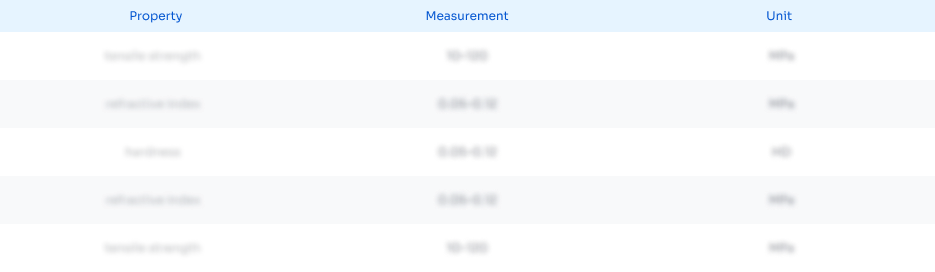
Abstract
Description
Claims
Application Information
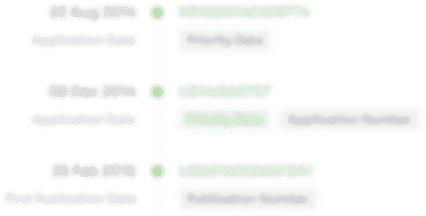
- Generate Ideas
- Intellectual Property
- Life Sciences
- Materials
- Tech Scout
- Unparalleled Data Quality
- Higher Quality Content
- 60% Fewer Hallucinations
Browse by: Latest US Patents, China's latest patents, Technical Efficacy Thesaurus, Application Domain, Technology Topic, Popular Technical Reports.
© 2025 PatSnap. All rights reserved.Legal|Privacy policy|Modern Slavery Act Transparency Statement|Sitemap|About US| Contact US: help@patsnap.com