Indoor telephone traffic accurate location method based on machine learning in cellular network
It is a machine learning and precise positioning technology, which can be used in location-based services, instruments, and wireless communication services. It can solve problems such as difficult positioning, dense high-rise buildings, and impact on positioning accuracy. Good training effect, high precision effect
- Summary
- Abstract
- Description
- Claims
- Application Information
AI Technical Summary
Problems solved by technology
Method used
Image
Examples
Embodiment Construction
[0022] The method for precise positioning of indoor traffic in a cellular network based on machine learning of the present invention will be further described below in conjunction with the accompanying drawings. figure 1 and figure 2 The overall method flow and typical application scenarios of the present invention are shown.
[0023] 1) Training module.
[0024] The base station system uses idle resources to gradually acquire a large number of UE signal parameters, including TOA, TDOA, AOA, RSS, etc. that may be acquired. Using these data to design a hybrid positioning algorithm, while considering non-line-of-sight identification and multipath suppression methods. Then, using prior information such as user habits and building locations, the location estimated by the hybrid positioning algorithm is corrected to obtain accurate location information. These position information and their corresponding original parameters are used as the training data of the machine learning a...
PUM
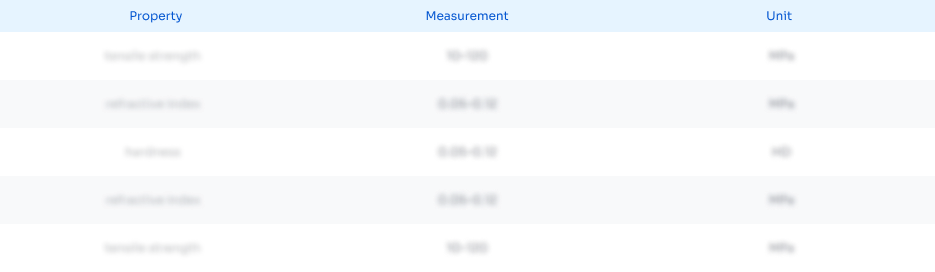
Abstract
Description
Claims
Application Information
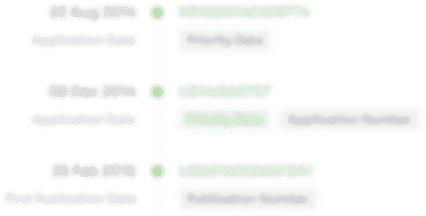
- R&D Engineer
- R&D Manager
- IP Professional
- Industry Leading Data Capabilities
- Powerful AI technology
- Patent DNA Extraction
Browse by: Latest US Patents, China's latest patents, Technical Efficacy Thesaurus, Application Domain, Technology Topic, Popular Technical Reports.
© 2024 PatSnap. All rights reserved.Legal|Privacy policy|Modern Slavery Act Transparency Statement|Sitemap|About US| Contact US: help@patsnap.com