Clustered adaptive window based hyperspectral image abnormality detection method
A hyperspectral image and anomaly detection technology, applied in image enhancement, image analysis, image data processing, etc., can solve problems such as hyperspectral image anomalies, achieve strong robustness and improve accuracy.
- Summary
- Abstract
- Description
- Claims
- Application Information
AI Technical Summary
Problems solved by technology
Method used
Image
Examples
specific Embodiment approach 1
[0013] Specific implementation mode one: the following combination figure 1 Describe this embodiment, the hyperspectral image anomaly detection method based on the cluster adaptive window described in this embodiment, the specific process of the hyperspectral image anomaly detection method is:
[0014] Step 1. Perform spectral dimension principal component analysis on hyperspectral images to generate spectral subspaces to achieve dimensionality reduction of spectral dimensions;
[0015] Step 2. Generate an adaptive window for each pixel to be detected; the generated adaptive window is a binary matrix, the center of the binary matrix coincides with the pixel to be detected, and a pixel with "1" in the matrix indicates that the hyperspectral image is the same The pixels in the homogeneous background area are used to calculate the statistical properties of the reference background. The pixels with "0" in the matrix indicate the pixels in the non-homogeneous background area in the...
specific Embodiment approach 2
[0019] Specific implementation mode two: the following combination figure 1 Describe this embodiment, this embodiment will further explain Embodiment 1, the specific process of performing spectral dimension principal component analysis on the hyperspectral image described in step 1 is:
[0020] Step 1-1, performing standardized transformation on the hyperspectral image matrix;
[0021] Step 1-2, obtaining the transformed correlation matrix;
[0022] Steps 1-3, obtaining the characteristic root of the correlation matrix, and determining the principal components of the hyperspectral image.
specific Embodiment approach 3
[0023] Specific implementation mode three: the following combination figure 1 Describe this embodiment, this embodiment will further explain Embodiment 1 or 2, the specific process of generating an adaptive window for each pixel to be detected as described in step 2 is:
[0024] Step 2-1. Generate a w×w adaptive window for each pixel to be tested, initialize the center of the adaptive window to "1", and initialize other areas to "0";
[0025] Step 2-2, using the Pearson criterion and the root mean square error minimization method to unmix the hyperspectral image, and determine the number of end members N;
[0026] Step 2-3, using the K-means clustering method to divide the hyperspectral image into N categories, and adding category labels to all pixels;
[0027] Steps 2-4: Compare the class labels of the pixels within the range of the adaptive window and the center point, and set the pixels in the adaptive window that are consistent with the label of the central pixel to "1" t...
PUM
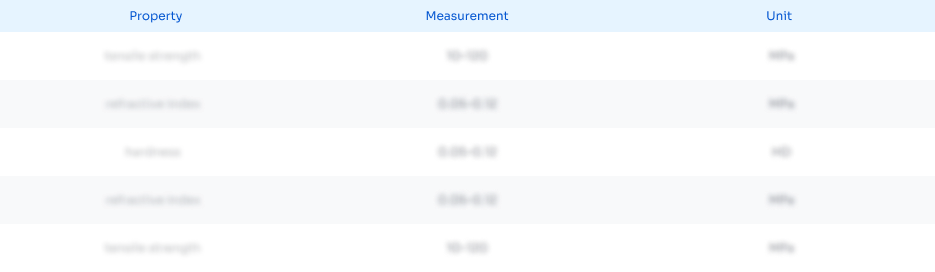
Abstract
Description
Claims
Application Information
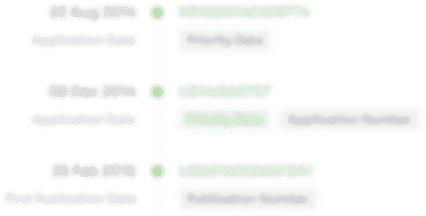
- R&D Engineer
- R&D Manager
- IP Professional
- Industry Leading Data Capabilities
- Powerful AI technology
- Patent DNA Extraction
Browse by: Latest US Patents, China's latest patents, Technical Efficacy Thesaurus, Application Domain, Technology Topic, Popular Technical Reports.
© 2024 PatSnap. All rights reserved.Legal|Privacy policy|Modern Slavery Act Transparency Statement|Sitemap|About US| Contact US: help@patsnap.com