A Convolutional Neural Network-Based Generation Method for Spatiotemporal Consistent Depth Map Sequences
A convolutional neural network, consistent technology, applied in the field of the generation of spatiotemporally consistent depth map sequences, can solve problems such as affecting the user's perception, flickering of virtual views, and ignoring
- Summary
- Abstract
- Description
- Claims
- Application Information
AI Technical Summary
Problems solved by technology
Method used
Image
Examples
specific Embodiment
[0126] Specific embodiments: the present invention is compared with existing methods in other collections on the public dataset NYU depth v2 and a dataset LYB3D-TV proposed by the inventor himself. Among them, the NYU depth v2 dataset consists of 795 training scenes and 654 test scenes, and each scene contains 30 consecutive frames of rgb images and their corresponding depth maps. The LYU3D-TV database is taken from some scenes of the TV series "Nirvana in Fire". We selected 5124 frames of pictures in 60 scenes and their manually-labeled depth maps as the training set, and 1278 frames of pictures in 20 scenes and their manual labels. The depth map is used as the test set. We compared the depth recovery accuracy of the proposed method with the following methods:
[0127] 1. Depth transfer: Karsch, Kevin, Ce Liu, and Sing Bing Kang. "Depth transfer: Depth extraction from video using non-parametric sampling." IEEE transactions on pattern analysis and machine intelligence 36.11(2...
PUM
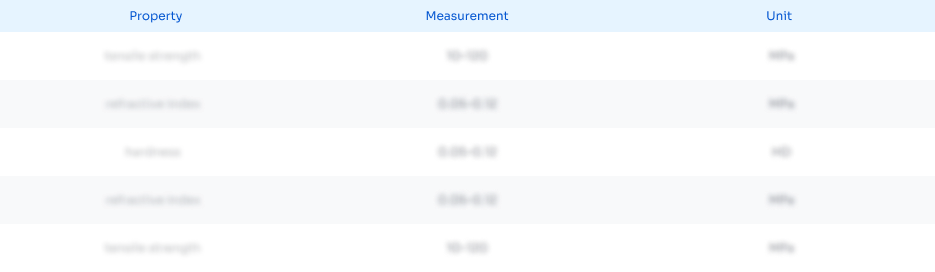
Abstract
Description
Claims
Application Information
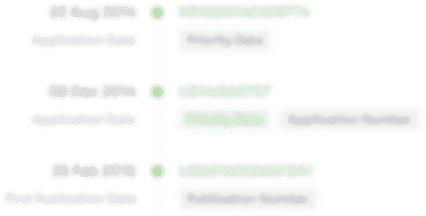
- R&D Engineer
- R&D Manager
- IP Professional
- Industry Leading Data Capabilities
- Powerful AI technology
- Patent DNA Extraction
Browse by: Latest US Patents, China's latest patents, Technical Efficacy Thesaurus, Application Domain, Technology Topic, Popular Technical Reports.
© 2024 PatSnap. All rights reserved.Legal|Privacy policy|Modern Slavery Act Transparency Statement|Sitemap|About US| Contact US: help@patsnap.com