Prediction method of monthly power consumption
A prediction method and electricity consumption technology, applied in data processing applications, instruments, energy industry, etc., can solve problems such as unconsidered connection, strong dependence on historical data, long learning time, etc.
- Summary
- Abstract
- Description
- Claims
- Application Information
AI Technical Summary
Problems solved by technology
Method used
Image
Examples
Embodiment
[0017] The Pearson correlation coefficient is a quantitative indicator describing the degree of correlation between two variables x and y, and its value is in the range of [-1, 1]. when r xy = 0, there is no correlation between x and y, which means that x and y are not correlated; when r xy >0, y increases with the increase of x, which means that x and y are positively correlated; when r xy xy When |=1, y can be expressed exactly by the linear function of variable x. The specific calculation formula is as follows: Table 1 shows the correlation strength corresponding to the value range of Pearson correlation coefficient.
[0018] Table 1 Pearson correlation coefficient value and correlation table
[0019]
[0020]
[0021] 1. Screening of industry indicators
[0022] Considering industry indicators as variable x and power consumption as variable y, the correlation coefficient between industry indicators and industry electricity consumption can be calculated, and the...
PUM
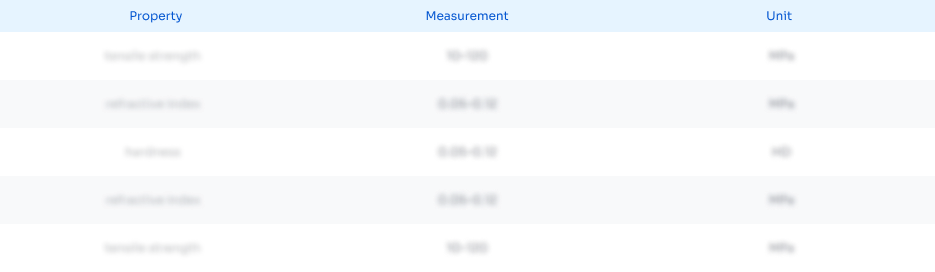
Abstract
Description
Claims
Application Information
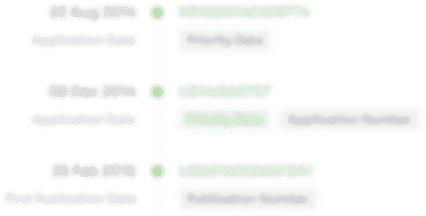
- R&D Engineer
- R&D Manager
- IP Professional
- Industry Leading Data Capabilities
- Powerful AI technology
- Patent DNA Extraction
Browse by: Latest US Patents, China's latest patents, Technical Efficacy Thesaurus, Application Domain, Technology Topic, Popular Technical Reports.
© 2024 PatSnap. All rights reserved.Legal|Privacy policy|Modern Slavery Act Transparency Statement|Sitemap|About US| Contact US: help@patsnap.com