Kernel extreme learning machine based quick traffic sign detecting method
An ultra-limited learning machine and traffic sign technology, applied in the field of image signal processing and pattern recognition, can solve the problems of difficult to meet real-time detection of traffic signs, slow detection speed, large search space, etc., to improve detection accuracy and detection. speed, improve detection speed, reduce the effect of search space
- Summary
- Abstract
- Description
- Claims
- Application Information
AI Technical Summary
Problems solved by technology
Method used
Image
Examples
Embodiment Construction
[0017] Attached below Figure 1-2 The present invention will be further described with specific embodiments.
[0018] Suppose there is a training data set TrainData and a set of test data sets TestData, the sample size of TrainData is N, each sample contains traffic signs, and the Ground-truth of traffic signs is known; the sample size of TestData is M, which does not contain traffic signs The identified samples; where the samples in TrainData and TestData belong to K categories;
[0019] A fast traffic sign detection method based on nuclear extreme learning machine, the flow chart is as follows figure 1 shown.
[0020] Step 1, read the original sample images in TrainData and TestData respectively, and scale the original images to a predefined size (36 different scales); calculate the NG features of the image at different scales, and standardize the gradient of each point to [0, 255 ]; binarize the NG features to obtain Btraindata and BtestData.
[0021] Step 2, use the 8*...
PUM
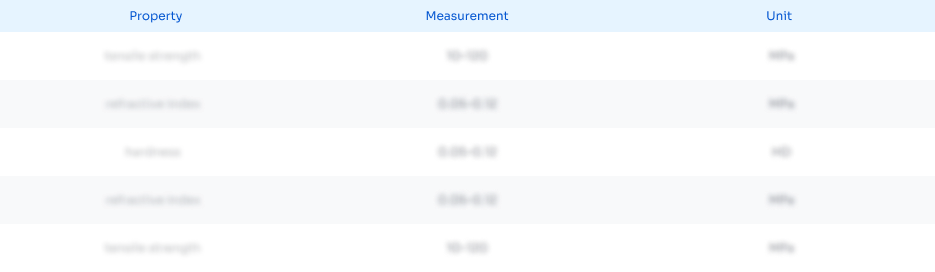
Abstract
Description
Claims
Application Information
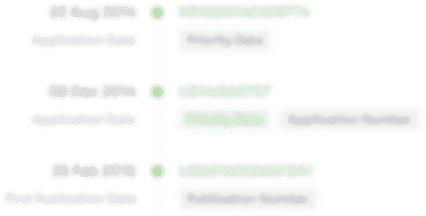
- R&D
- Intellectual Property
- Life Sciences
- Materials
- Tech Scout
- Unparalleled Data Quality
- Higher Quality Content
- 60% Fewer Hallucinations
Browse by: Latest US Patents, China's latest patents, Technical Efficacy Thesaurus, Application Domain, Technology Topic, Popular Technical Reports.
© 2025 PatSnap. All rights reserved.Legal|Privacy policy|Modern Slavery Act Transparency Statement|Sitemap|About US| Contact US: help@patsnap.com