Rolling bearing fault diagnosis method
A rolling bearing and fault diagnosis technology, applied in mechanical bearing testing, instrument, character and pattern recognition, etc., can solve problems such as nonlinearity, non-stationary signal uncertainty, different decomposition results, difficult selection of wavelet bases, etc.
- Summary
- Abstract
- Description
- Claims
- Application Information
AI Technical Summary
Problems solved by technology
Method used
Image
Examples
Embodiment Construction
[0086] The specific implementation manners of the present invention will be further described in detail below in conjunction with the accompanying drawings and embodiments. The following examples are used to illustrate the present invention, but are not intended to limit the scope of the present invention.
[0087] In order to overcome the deficiencies in the prior art, the rolling bearing fault diagnosis method based on the convolutional neural network and support vector regression of the present invention first adopts the convolutional neural network to learn the essential characteristics of the training sample data, and then adopts the support vector regression classification method to test the samples Classification and identification are carried out to determine the category of rolling bearing fault conditions, so as to improve the accuracy and effectiveness of rolling bearing fault diagnosis.
[0088] Convolutional Neural Network (CNN for short) has a powerful function e...
PUM
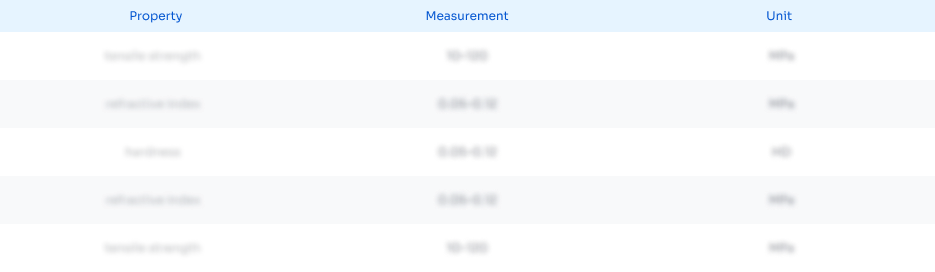
Abstract
Description
Claims
Application Information
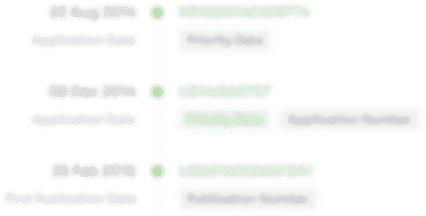
- R&D Engineer
- R&D Manager
- IP Professional
- Industry Leading Data Capabilities
- Powerful AI technology
- Patent DNA Extraction
Browse by: Latest US Patents, China's latest patents, Technical Efficacy Thesaurus, Application Domain, Technology Topic.
© 2024 PatSnap. All rights reserved.Legal|Privacy policy|Modern Slavery Act Transparency Statement|Sitemap