Particle swarm optimization algorithm, multi-computer parallel processing method and system
A particle swarm optimization and particle swarm technology, which is applied in various digital computer combinations, calculations, calculation models, etc., can solve the problem of optimization algorithms falling into local optimum, achieve the effect of improving accuracy and efficiency, and expanding coverage
- Summary
- Abstract
- Description
- Claims
- Application Information
AI Technical Summary
Problems solved by technology
Method used
Image
Examples
Embodiment 1
[0055] Such as figure 1 and figure 2 As shown, the present embodiment 1 provides a particle swarm optimization algorithm, including:
[0056] Step S1, initialize the generalized interval particle swarm;
[0057] Step S2, calculating the initial fitness value of each particle; and
[0058] Step S3, iteratively updating the velocity and position of the particle swarm until the global optimal solution is output.
[0059] Specifically, the method for initializing the generalized interval particle swarm in step S1 includes:
[0060] Suppose there are m particles in this particle swarm, and each particle is a generalized interval. The initial position and initial velocity expressions of each particle are as follows:
[0061] Initial position expression:
[0062] in, is the lower bound of the initial position of the i-th particle, is an upper bound on the initial position of the i-th particle; and
[0063] Initial velocity expression:
[0064] in, is the lower bound...
Embodiment 2
[0089] Such as image 3 As shown, on the basis of Embodiment 1, Embodiment 2 also provides a multi-computer parallel processing method.
[0090] Adopt the particle swarm optimization algorithm as described in embodiment 1 to obtain global optimal solution by main frame and some extensions, namely
[0091] Each extension is suitable for calculating the position and velocity of the assigned particle at each moment, and record the best position of the particle, and transmit the calculation result to the host;
[0092] The host is suitable for collecting the optimal position, current position and speed of each particle transmitted by each extension, calculating the position of the global optimal particle, and transmitting this information to each extension for each extension to determine the speed of the particle it belongs to.
Embodiment 3
[0094] Such as image 3 As shown, on the basis of embodiment 1, this embodiment 3 also provides a multi-computer parallel computing system applying the particle swarm optimization algorithm as described in embodiment 1.
[0095] The multi-computer parallel computing system includes: a mainframe and several extensions, wherein
[0096] Each extension is suitable for calculating the position and velocity of the assigned particle at each moment, and record the best position of the particle, and transmit the calculation result to the host;
[0097] The host is suitable for collecting the optimal position, current position and speed of each particle transmitted by each extension, calculating the position of the global optimal particle, and transmitting this information to each extension for each extension to determine the speed of the particle it belongs to.
[0098] On the basis of embodiment 2 and embodiment 3, by Figure 4 It can be seen that compared with the traditional part...
PUM
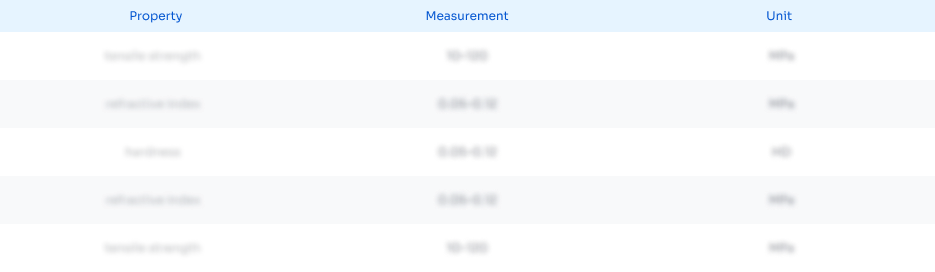
Abstract
Description
Claims
Application Information
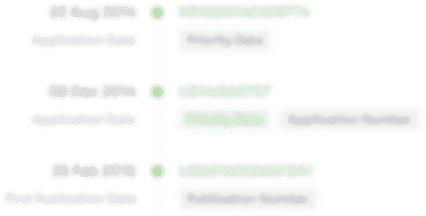
- R&D
- Intellectual Property
- Life Sciences
- Materials
- Tech Scout
- Unparalleled Data Quality
- Higher Quality Content
- 60% Fewer Hallucinations
Browse by: Latest US Patents, China's latest patents, Technical Efficacy Thesaurus, Application Domain, Technology Topic, Popular Technical Reports.
© 2025 PatSnap. All rights reserved.Legal|Privacy policy|Modern Slavery Act Transparency Statement|Sitemap|About US| Contact US: help@patsnap.com