Spectral clustering method for automatically determining number of clusters based on neighboring point method
A technology for automatically determining and adjacent points, applied in the field of spectral clustering algorithms, can solve problems such as reducing space complexity and high space complexity
- Summary
- Abstract
- Description
- Claims
- Application Information
AI Technical Summary
Problems solved by technology
Method used
Image
Examples
Embodiment Construction
[0067] The present invention will be further described below in conjunction with the accompanying drawings.
[0068] refer to Figure 1 to Figure 5 , a spectral clustering method for automatically determining the number of clusters based on the neighboring point method, comprising the following steps:
[0069] 1) Data preprocessing, by analyzing the actual data set, we can see that some dimensions of some data sets are much larger than other dimensions of the data set, and the difference between the values of these dimensions is large, which leads to the importance of other dimensions. Sexuality may be muted or even ignored. In the absence of information about the importance of each dimension of the dataset, we normalized all dimensions of the dataset, the specific process is as follows:
[0070] Input data set, for each dimension x of the data set 1 ,...,x n ∈R m Do normalization processing, that is, the value of the j-th dimension after the i-th data is processed is: ...
PUM
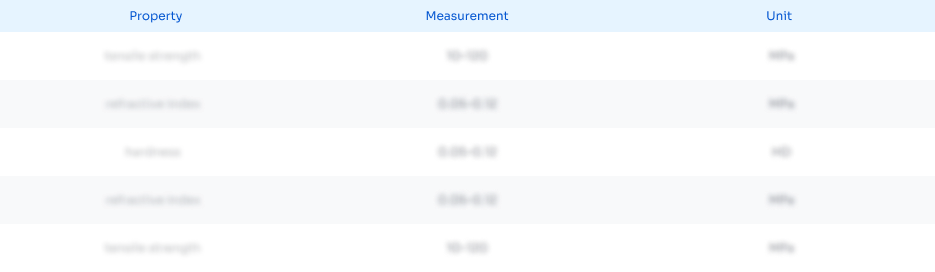
Abstract
Description
Claims
Application Information
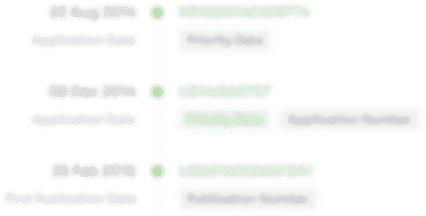
- R&D Engineer
- R&D Manager
- IP Professional
- Industry Leading Data Capabilities
- Powerful AI technology
- Patent DNA Extraction
Browse by: Latest US Patents, China's latest patents, Technical Efficacy Thesaurus, Application Domain, Technology Topic, Popular Technical Reports.
© 2024 PatSnap. All rights reserved.Legal|Privacy policy|Modern Slavery Act Transparency Statement|Sitemap|About US| Contact US: help@patsnap.com