Multilevel model cloth defect detection method and system
A detection method and multi-level technology, applied in the field of pattern recognition, can solve the problems of low timeliness, low efficiency of manual inspection of defects, low accuracy, etc., to achieve a large amount of calculation, improve accuracy and real-time performance, and ensure accuracy Effect
- Summary
- Abstract
- Description
- Claims
- Application Information
AI Technical Summary
Problems solved by technology
Method used
Image
Examples
specific Embodiment approach
[0047] The specific implementation method is: select 3000 sample images with a size of 227×227 as the learning set L1, including 1000 normal cloth images and 2000 defect images, including 500 holes, foreign objects, oil stains and crease defect images each. L1 is divided into training set L1 according to 4:1 1 and validation set L1 2 ; Compute L1 1 The 4 eigenvalues of the GLCM in the 4 directions of the image, the 4 eigenvalues are energy, entropy, contrast and inverse moment, and the obtained eigenvector V 11 Input the support vector machine (SVM) binary classification model for training, after a certain number of iterations, calculate L1 2 The 4 eigenvalues of the GLCM in the 4 directions of the image, the 4 eigenvalues are energy, entropy, contrast and inverse moment, and the obtained eigenvector V 12 Input the support vector machine (SVM) binary classification model for testing, if the test accuracy rate does not meet the requirements, continue training, and fi...
PUM
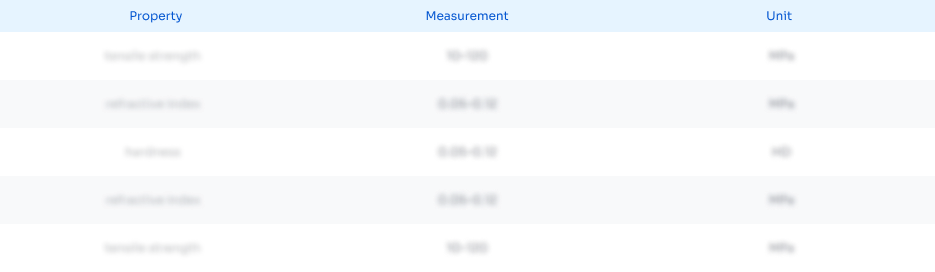
Abstract
Description
Claims
Application Information
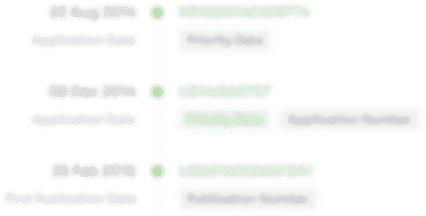
- Generate Ideas
- Intellectual Property
- Life Sciences
- Materials
- Tech Scout
- Unparalleled Data Quality
- Higher Quality Content
- 60% Fewer Hallucinations
Browse by: Latest US Patents, China's latest patents, Technical Efficacy Thesaurus, Application Domain, Technology Topic, Popular Technical Reports.
© 2025 PatSnap. All rights reserved.Legal|Privacy policy|Modern Slavery Act Transparency Statement|Sitemap|About US| Contact US: help@patsnap.com