A real-time super-resolution reconstruction method
A super-resolution reconstruction and resolution technology, applied in the field of image and video, can solve the problems of inability to realize real-time super-resolution reconstruction and low reconstruction efficiency, and achieve the effect of reducing the number of dictionary atoms, low cost, and improving computing efficiency.
- Summary
- Abstract
- Description
- Claims
- Application Information
AI Technical Summary
Problems solved by technology
Method used
Image
Examples
Embodiment 1
[0049] See figure 1 , figure 1 It is a schematic diagram of a real-time super-resolution reconstruction method provided by an embodiment of the present invention. The method includes the following steps:
[0050] Step 1. Form a second resolution image according to the first resolution image processing, and determine a resolution dictionary from the first resolution image and the second resolution image;
[0051] Step 2: Perform block processing on the image to be reconstructed to form multiple image blocks to be reconstructed;
[0052] Step 3. Set the information volume threshold and determine whether the information volume of the image block to be reconstructed is greater than the information volume threshold; if so, perform image reconstruction on the image block to be reconstructed according to the resolution dictionary to form a first sub area;
[0053] Step 4. Perform image stitching on the first sub-region to obtain a super-resolution reconstructed image.
[0054] Among them, fo...
Embodiment 2
[0090] This embodiment provides a detailed description of the technical solution of the present invention on the basis of the foregoing embodiment. Specifically, the method includes:
[0091] Step 1: Using a large number of samples of high-resolution images (ie, the first resolution image), the high-resolution images are subjected to blur processing and N-fold down-sampling according to the modified degradation model to obtain the corresponding low-resolution images (ie, the first resolution image). Two-resolution image) sample.
[0092] Step 2: For the low-resolution image obtained in step 1, the image features are extracted through the feature extraction algorithm to obtain the high-resolution feature information X of the space target s (I.e. first resolution feature information) and low resolution feature information Y s (Ie the second resolution feature information).
[0093] Step 3: Use the K-SVD algorithm to jointly train the feature information to obtain a high-resolution dic...
Embodiment approach
[0110] S1: Perform block segmentation on a large number of low-resolution image samples to obtain image blocks;
[0111] S2: Use an edge extraction algorithm to extract edge information of low-resolution image blocks, and count the information volume of each low-resolution image block and the distribution of information volume of all low-resolution image blocks;
[0112] S3: Select the value with the highest amount of information in the low-resolution image block, obtain the pixel value of the low-resolution image block as F1, f=F1 / 4, then f*40% <= threshold <=f*60%, take several representative thresholds in this range, for example, you can take the following representative thresholds: f*40%, f*45%, f*50%, f*55%, f*60%, calculate each The learning-based sparse representation of each threshold point corresponds to the reconstruction time of the image super-resolution reconstruction algorithm and the resolution of the reconstructed image, which can be judged according to subjective ev...
PUM
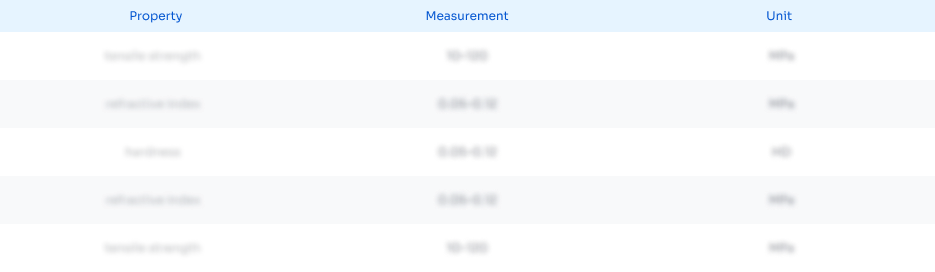
Abstract
Description
Claims
Application Information
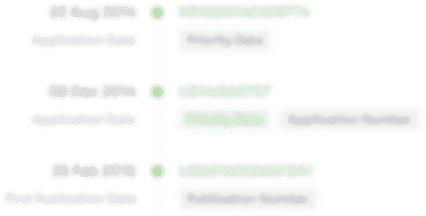
- R&D Engineer
- R&D Manager
- IP Professional
- Industry Leading Data Capabilities
- Powerful AI technology
- Patent DNA Extraction
Browse by: Latest US Patents, China's latest patents, Technical Efficacy Thesaurus, Application Domain, Technology Topic, Popular Technical Reports.
© 2024 PatSnap. All rights reserved.Legal|Privacy policy|Modern Slavery Act Transparency Statement|Sitemap|About US| Contact US: help@patsnap.com