Human Action Recognition Method Based on Convolutional Neural Network Feature Coding
A convolutional neural network and human action recognition technology, applied in the field of image processing, can solve problems such as low accuracy, large amount of calculation, and slow speed, so as to improve the accuracy of recognition, stabilize vector features, and reduce the amount of calculation Effect
- Summary
- Abstract
- Description
- Claims
- Application Information
AI Technical Summary
Problems solved by technology
Method used
Image
Examples
Embodiment 1
[0039]At present, human action recognition has a wide range of application values in life. In terms of scientific research, there are also many studies on human action recognition. Existing human action recognition methods mainly include methods based on template matching, neural networks, and spatiotemporal features. The above method has high time complexity, large amount of calculation, poor real-time performance, easy to be blocked, requires a large memory, complex to implement, low recognition rate, and is easily affected by environmental conditions in the realization of human action recognition methods, which leads to this problem. When dealing with a large amount of complex background data, such methods reduce the accuracy of human action recognition due to their weak robustness. In view of this current situation, the present invention has carried out innovation and research, and proposed a human action recognition method based on convolutional neural network feature ...
Embodiment 2
[0059] The human action recognition method based on convolutional neural network feature coding is the same as in embodiment 1. In step (5) of the present invention, the convolution features of the spatial direction video and the convolution features of the action direction optical flow graph are respectively subjected to local feature accumulation coding to obtain local features. Feature accumulation descriptor, including the following steps:
[0060] (5a) Accumulate and superimpose the pixel values at the same position in the 512 6×6 pixel size convolution feature maps obtained from each image in the human action video image in the spatial direction, and 36 512-dimensional local feature accumulation descriptors can be obtained , the local feature accumulation descriptor of a video can be expressed as n × (36 × 512), where n represents the number of frames of the video;
[0061] (5b) 512 6×6-pixel convolutional feature maps obtained for each optical flow map in the action d...
Embodiment 3
[0064] The human action recognition method based on convolutional neural network feature coding is the same as that in Embodiment 1-2. In the step (6) of the present invention, principal component analysis (PCA) is used to reduce the dimension and whiten the local feature accumulation descriptors of the spatial direction and the action direction respectively. Proceed as follows:
[0065] (6a) Use principal component analysis PCA to perform dimensionality reduction and whitening processing on the local feature accumulation descriptor in the spatial direction;
[0066] (6a1) Randomly extract 10,000 local feature cumulative descriptors from the encoded local feature cumulative descriptors, expressed as {x 1 ,...,x i ,...,x m}, as the input data for PCA processing, where i∈[1,m], m is the number of cumulative descriptors of local features;
[0067] (6a2) Calculate the mean value of each local feature cumulative descriptor according to the following formula
[0068]
[006...
PUM
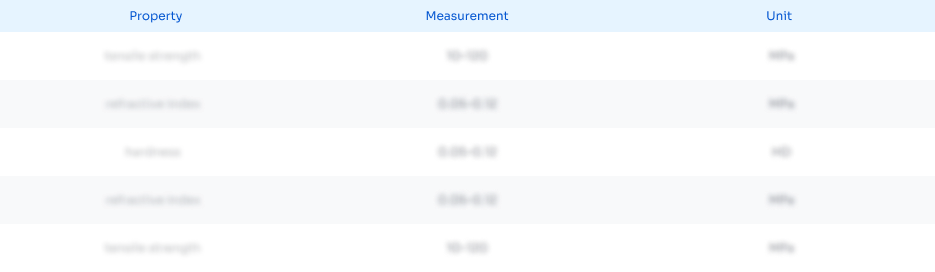
Abstract
Description
Claims
Application Information
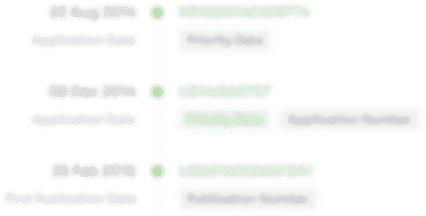
- R&D
- Intellectual Property
- Life Sciences
- Materials
- Tech Scout
- Unparalleled Data Quality
- Higher Quality Content
- 60% Fewer Hallucinations
Browse by: Latest US Patents, China's latest patents, Technical Efficacy Thesaurus, Application Domain, Technology Topic, Popular Technical Reports.
© 2025 PatSnap. All rights reserved.Legal|Privacy policy|Modern Slavery Act Transparency Statement|Sitemap|About US| Contact US: help@patsnap.com