Realization method of atrial fibrillation detection based on deep convolutional neural network
A neural network and deep convolution technology, applied in the field of atrial fibrillation detection, can solve the problem of not finding the successful application of deep convolutional neural network, and achieve the effect of improving efficiency and accuracy
- Summary
- Abstract
- Description
- Claims
- Application Information
AI Technical Summary
Problems solved by technology
Method used
Image
Examples
specific Embodiment approach 1
[0023] Specific Embodiment 1: This embodiment provides an implementation method of atrial fibrillation detection based on a deep convolutional neural network, in order to make the original one-dimensional ECG signal suitable for processing a convolutional neural network structure in the form of two-dimensional data , it is necessary to properly transform the one-dimensional ECG signal to meet the requirements of the deep convolutional neural network for the input signal. In addition, the realization method of atrial fibrillation detection based on the deep convolutional neural network provided in this embodiment, the deep convolutional neural network is constructed based on Caffe, one of the most popular deep learning frameworks at present. The specific implementation steps are as follows:
[0024] (1) Use ECG acquisition equipment to collect continuous ECG data of a single lead of the patient, and the doctor will mark each beat of the ECG data whether there is atrial fibrilla...
specific Embodiment approach 2
[0044] Specific embodiment two: This embodiment provides a method for detecting atrial fibrillation based on static wavelet transform combined with deep convolutional neural network, such as figure 2 As shown, the specific steps are as follows:
[0045] Step (1): read in the records in the MIT-BIH atrial fibrillation database.
[0046] Step (2): Carry out data segmentation for these ECG records. The duration of each data segment is 5 seconds. Since the sampling frequency of the MIT-BIH atrial fibrillation database is 250Hz, each 5-second data segment contains 1250 Sampling point. Set the sample category label for each 5-second data segment according to the annotation file of the MIT-BIH atrial fibrillation database. The basis for setting the label is: if the proportion of the number of atrial fibrillation beats in each 5-second data segment to the entire data segment is ≥ 50%, the data segment is marked as an atrial fibrillation segment, otherwise it is a non-atrial fibrill...
specific Embodiment approach 3
[0058] Specific Embodiment Three: This embodiment provides a method for detecting atrial fibrillation based on short-time Fourier transform combined with a deep convolutional neural network, such as image 3 As shown, the specific steps are as follows:
[0059] Step (1): read in the records in the MIT-BIH atrial fibrillation database.
[0060] Step (2): Carry out data segmentation for these ECG records. The duration of each data segment is 5 seconds. Since the sampling frequency of the MIT-BIH atrial fibrillation database is 250Hz, each 5-second data segment contains 1250 Sampling point. Set the sample category label for each 5-second data segment according to the annotation file of the MIT-BIH atrial fibrillation database. The basis for setting the label is: if the proportion of the number of atrial fibrillation beats in each 5-second data segment to the entire data segment is ≥ 50%, the data segment is marked as an atrial fibrillation segment, otherwise it is a non-atrial ...
PUM
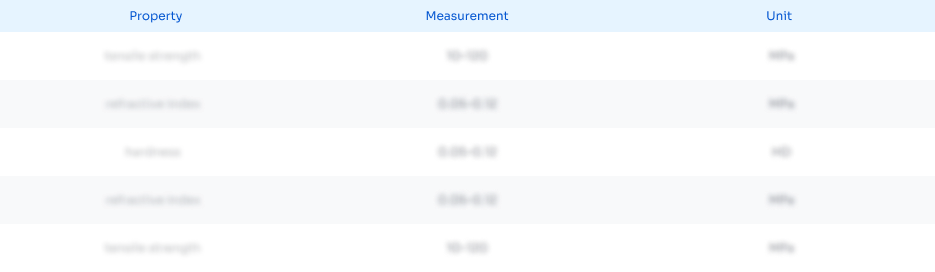
Abstract
Description
Claims
Application Information
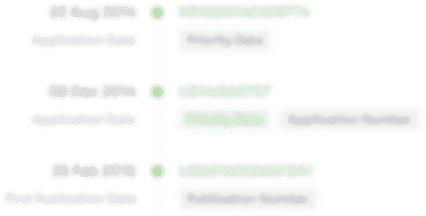
- R&D Engineer
- R&D Manager
- IP Professional
- Industry Leading Data Capabilities
- Powerful AI technology
- Patent DNA Extraction
Browse by: Latest US Patents, China's latest patents, Technical Efficacy Thesaurus, Application Domain, Technology Topic, Popular Technical Reports.
© 2024 PatSnap. All rights reserved.Legal|Privacy policy|Modern Slavery Act Transparency Statement|Sitemap|About US| Contact US: help@patsnap.com