K distribution sea clutter shape parameter estimation method based on neural network
A shape parameter and neural network technology, applied in the field of signal processing, can solve problems such as difficulty in obtaining sea clutter data, and achieve high precision, good robustness, and high efficiency
- Summary
- Abstract
- Description
- Claims
- Application Information
AI Technical Summary
Problems solved by technology
Method used
Image
Examples
Embodiment 1
[0028] Improving the accuracy of sea clutter shape parameter estimation in the presence of abnormal samples can better reflect the actual situation of sea conditions, reduce the false alarm rate and missed detection rate in sea clutter target detection, and improve detection performance. Efficient and fast estimation of sea clutter shape parameters under sample conditions is an urgent problem to be solved. The existing estimation methods estimate the sea clutter shape parameters by combining all the amplitude characteristics, ignoring the influence of abnormal samples. In view of this shortcoming, the present invention conducts research and discussion, and proposes a neural network-based K Distributed sea clutter shape parameter estimation method, see figure 1 , including the following steps:
[0029] (1) Acquisition of ideal pure sea clutter data: under the condition of ensuring power normalization, the simulation software is used to generate multiple sets of independent K-d...
Embodiment 2
[0040] The K distribution sea clutter shape parameter estimation method based on neural network is the same as embodiment 1, and step (1) acquires the ideal pure sea clutter data, including the following steps:
[0041] 1a) The probability density function ρ of the K distribution x Expressed as:
[0042]
[0043] Among them, v represents the shape parameter (v>0), b represents the scale parameter (b>0), K v (·) represents the v-order modified Bessel function of the second kind.
[0044] Get K distribution power E(x 2 )for:
[0045] E(x 2 ) = vb.
[0046] 1b) at In the case of , 10 groups of clutter samples are generated for each shape parameter with an interval of 0.01 and a range of 0.1-5, and the representation of the clutter sample sequence Z is as follows:
[0047]
[0048] in is the clutter sample sequence with a as the shape parameter and b as the group number, a(a=0.1,0.11,,5) represents the shape parameter, b(b=1,2,…,10) represents the group number, z ...
Embodiment 3
[0052] The K distribution sea clutter shape parameter estimation method based on neural network is the same as embodiment 1-2, and step (2) trains neural network, comprising the following steps:
[0053] 2a) The K distribution is used to simulate each group of amplitude sequences in the clutter data Sort the elements in the sequence from small to large to obtain multiple sets of sequence sequences, and divide the sequence sequence into 26 subsequences evenly. If the division cannot be performed accurately, the default element in the first subsequence is the least , and take the last element of each subsequence in the first 25 subsequences as the value of the quantile point, so that the magnitude values of the 25 quantile points are taken in turn as the input of the neural network; the hidden layer is designed to be 50 nodes; the expectation The output is the shape parameter value a corresponding to the clutter sample group. Such a set of inputs and outputs constitutes a sa...
PUM
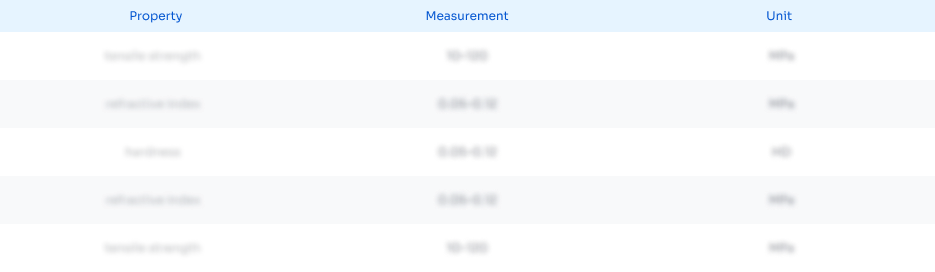
Abstract
Description
Claims
Application Information
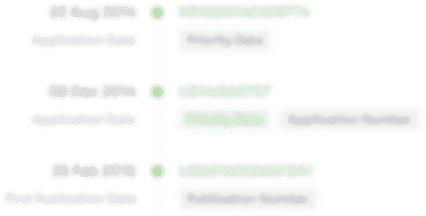
- R&D Engineer
- R&D Manager
- IP Professional
- Industry Leading Data Capabilities
- Powerful AI technology
- Patent DNA Extraction
Browse by: Latest US Patents, China's latest patents, Technical Efficacy Thesaurus, Application Domain, Technology Topic, Popular Technical Reports.
© 2024 PatSnap. All rights reserved.Legal|Privacy policy|Modern Slavery Act Transparency Statement|Sitemap|About US| Contact US: help@patsnap.com