A Resource Scheduling Method for Detecting Advanced Persistent Threats Based on Reinforcement Learning
An advanced persistent threat and resource scheduling technology, applied in the field of resource scheduling to detect advanced persistent threats, can solve problems such as restricting the application of reinforcement learning algorithms and decreasing the learning speed, and achieve the goal of speeding up learning, speeding up cognition, and improving data privacy performance. Effect
- Summary
- Abstract
- Description
- Claims
- Application Information
AI Technical Summary
Problems solved by technology
Method used
Image
Examples
Embodiment Construction
[0033] The technical solution of the present invention is further described below in conjunction with the examples, but the scope of protection is not limited to the description.
[0034] A resource scheduling method for detecting advanced persistent threats based on reinforcement learning. The specific implementation steps are as follows:
[0035] Step 1: The defense system utilizes S M =Computing resources such as 16 CPUs detect advanced persistent threats (APT) in computers or cloud storage systems, utilize Computing resources such as a CPU detect the i-th cloud storage device at time k, where 1≤i≤D, D=4. The defense system detects that the resource allocation vectors of D cloud storage devices are The optional action ranges are: The number of optional actions is |Δ D |.
[0036] Step 2: The defense system observes the number of CPU and other computing resources used by APT attacks on D cloud storage devices at the last moment As the state s of the system at the c...
PUM
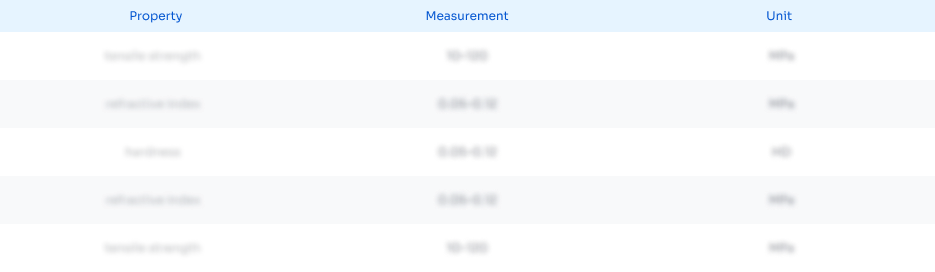
Abstract
Description
Claims
Application Information
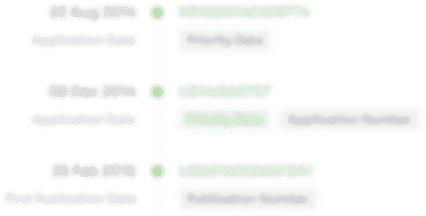
- R&D
- Intellectual Property
- Life Sciences
- Materials
- Tech Scout
- Unparalleled Data Quality
- Higher Quality Content
- 60% Fewer Hallucinations
Browse by: Latest US Patents, China's latest patents, Technical Efficacy Thesaurus, Application Domain, Technology Topic, Popular Technical Reports.
© 2025 PatSnap. All rights reserved.Legal|Privacy policy|Modern Slavery Act Transparency Statement|Sitemap|About US| Contact US: help@patsnap.com