CNN-based automatic identification method of lepidopteron types
A technology for lepidopteran insects and species is applied in the field of automatic identification of lepidopteran insects, and the image acquisition method is simple, easy to operate, and has good fault tolerance.
- Summary
- Abstract
- Description
- Claims
- Application Information
AI Technical Summary
Problems solved by technology
Method used
Image
Examples
example 1
[0047] 1. Use the matting function module attached to "Light and Shadow Magic Hand" or the GrabCut+Lazy Snapping tool to complete from Figure 1 to Figure 2 The background removal works and sets the background to black.
[0048] 2. Obtain the maximum bounding box of the insect image from the insect image after background removal.
[0049] 3. Check the longest side of the largest bounding box, if > 224, then perform proportional reduction to make the longest side ≤ 224.
[0050] 4. With the largest bounding box as the center, cut out a 227×227 image as the result of preprocessing.
[0051] 5. After all the training samples are pre-processed above, input them to the AlexNet network pre-trained by ImageNet (such as Figure 4 ), taking the result of layer 7 (a vector of length 4096) as the feature vector.
[0052] 6. Use the feature vector extracted from the training sample set to train χ 2 Kernel SVM classifier, each type of insect corresponds to a SVM model;
[0053] 7. The...
example 2
[0055] 1. Use the matting function module attached to "Light and Shadow Magic Hand" or the GrabCut+Lazy Snapping tool to complete from Figure 1 to Figure 2 The background removal works and sets the background to black.
[0056] 2. Obtain the maximum bounding box of the insect image from the insect image after background removal.
[0057] 3. Check the longest side of the largest bounding box, if > 224, then perform proportional reduction to make the longest side ≤ 224.
[0058] 4. With the largest bounding box as the center, cut out a 227×227 image as the result of preprocessing.
[0059] 5. After all the training samples are pre-processed above, they are input to the VGG16 network pre-trained by ImageNet, and the result of the second fully connected layer (vector with a length of 4096) is used as the feature vector.
[0060] 6. Use the feature vector extracted from the training sample set to train χ 2 Kernel SVM classifier, each type of insect corresponds to a SVM model; ...
example 3
[0063] 1. Use the matting function module attached to "Light and Shadow Magic Hand" or the GrabCut+Lazy Snapping tool to complete from Figure 1 to Figure 2 The background removal works and sets the background to black.
[0064] 2. Obtain the maximum bounding box of the insect image from the insect image after background removal.
[0065] 3. Check the longest side of the largest bounding box, if > 224, then perform proportional reduction to make the longest side ≤ 224.
[0066] 4. With the largest bounding box as the center, cut out a 227×227 image as the result of preprocessing.
[0067] 5. After all the training samples are pre-processed above, input them to the AlexNet network pre-trained by ImageNet (such as Figure 4 ), to carry out end-to-end training on the AlexNet network, because the CNN network parameters are fine-tuned to make it suitable for insect recognition, so the learning rate of the first 7 layers, including 5 convolutional layers and 2 fully connected laye...
PUM
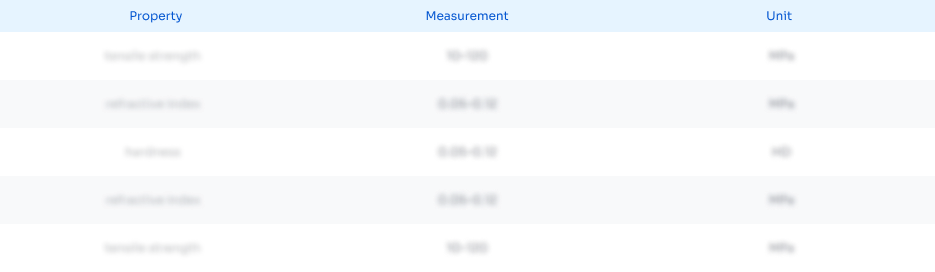
Abstract
Description
Claims
Application Information
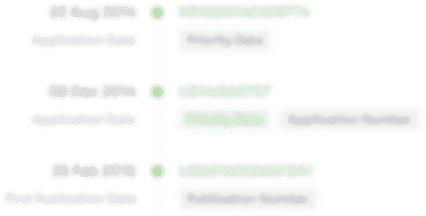
- R&D
- Intellectual Property
- Life Sciences
- Materials
- Tech Scout
- Unparalleled Data Quality
- Higher Quality Content
- 60% Fewer Hallucinations
Browse by: Latest US Patents, China's latest patents, Technical Efficacy Thesaurus, Application Domain, Technology Topic, Popular Technical Reports.
© 2025 PatSnap. All rights reserved.Legal|Privacy policy|Modern Slavery Act Transparency Statement|Sitemap|About US| Contact US: help@patsnap.com