Coal worker disease incidence rate prediction method based on radial primary function neural network combination model
A technology that combines models and prediction methods, applied in biological neural network models, neural learning methods, data processing applications, etc., can solve problems such as the gap between prediction results and actual requirements, and achieve clear algorithm derivation, accurate prediction results, and learning accuracy high effect
- Summary
- Abstract
- Description
- Claims
- Application Information
AI Technical Summary
Problems solved by technology
Method used
Examples
specific Embodiment approach 1
[0020] The method for predicting the morbidity of coal workers based on the radial basis function neural network combination model of the present embodiment, the described method is realized through the following steps:
[0021] Step 1. Collect and preprocess the data on the pathogenic factors of pneumoconiosis. The pathogenic factors include: dust type E related to dust toxicity, dust concentration in the working environment C, and dust exposure time T. The relationship between the three pathogenic factors is: P =F(E,C,T);
[0022] Step 2, modeling the BP neural network, determining the number of layers of the input and output layers, hidden layers and output layers, and adjusting thresholds and weights;
[0023] Step 3, using the genetic algorithm to optimize the BP neural network to obtain the optimal network initial weight and network threshold;
[0024] Step 4: Carry out result prediction and optimize the prediction result;
[0025] Step five, forming a prediction resul...
specific Embodiment approach 2
[0031] The difference from the specific embodiment 1 is that the method for predicting the morbidity of coal workers based on the radial basis function neural network combination model in this embodiment, the process of collecting the data of the pathogenic factors of pneumoconiosis described in step 1 is that the experiment and field investigation are used Data required by the mobile phone.
specific Embodiment approach 3
[0032] The difference from the specific embodiment 1 or 2 is that the method for predicting the morbidity of coal workers based on the radial basis function neural network combination model of this embodiment, the BP neural network described in step 2 is modeled, and the entry and exit layers, hidden Including layers and the number of layers of the output layer, in the process of adjusting the threshold and weight, the involved
[0033] (1) Modeling the BP neural network is specifically:
[0034] Suppose the actual measured value of the predicted incidence probability of coal worker pneumoconiosis is X=(x 1 , x 2 , x 3 ... x n ), there are m different models for prediction, and m≥2, the weights in the combined model are vector w=(w 1 ,w 2 ......w m ), the prediction value of the jth prediction model is Then the predicted value of period t in the combined forecasting model is In the formula, t=1, 2, 3, 4...n, the actual observation value of the tth period is X t , th...
PUM
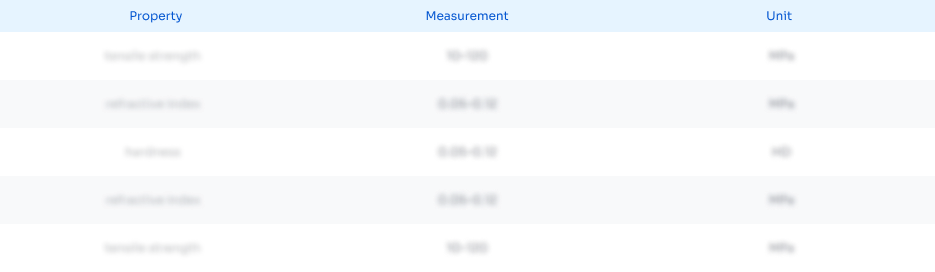
Abstract
Description
Claims
Application Information
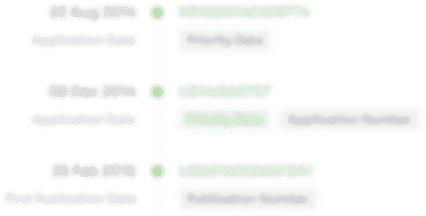
- R&D Engineer
- R&D Manager
- IP Professional
- Industry Leading Data Capabilities
- Powerful AI technology
- Patent DNA Extraction
Browse by: Latest US Patents, China's latest patents, Technical Efficacy Thesaurus, Application Domain, Technology Topic, Popular Technical Reports.
© 2024 PatSnap. All rights reserved.Legal|Privacy policy|Modern Slavery Act Transparency Statement|Sitemap|About US| Contact US: help@patsnap.com