Neural network compound learning control method for hypersonic vehicle re-entry stage
A hypersonic and neural network technology, applied in the direction of adaptive control, general control system, control/regulation system, etc., can solve problems such as poor precision, improve control precision, reduce conservatism, improve learning accuracy and rapidity Effect
- Summary
- Abstract
- Description
- Claims
- Application Information
AI Technical Summary
Problems solved by technology
Method used
Image
Examples
Embodiment Construction
[0027] refer to figure 1 . The specific steps of the hypersonic vehicle re-entry section neural network compound learning control method of the present invention are as follows:
[0028] (a) Establish the dynamic model of hypersonic vehicle re-entry section:
[0029]
[0030]
[0031] The dynamic model contains state variables X = [v, ω] T and control input U=M c , where v=[α β σ] T is the attitude angle vector, α, β, σ represent the angle of attack, sideslip angle and tilt angle respectively; ω=[p q r] T is the attitude angular rate vector, p, q, r represent the rolling, pitching and yaw angular rates respectively; M c =[M x m y m z ] T Indicates the control torque of the system;
[0032] (b) Define X=[x 1 x 2 ] T , x 1 =v,x 2 =ω. Then the attitude control model can be expressed as:
[0033]
[0034] where g 1 (x 1 )=R(·), f 2 (x 2 )=-I -1 ΩIω,g 2 (x 2 ) = I -1 .
[0035] (c) Define the attitude angle tracking error e 1 =x 1 -y d ;...
PUM
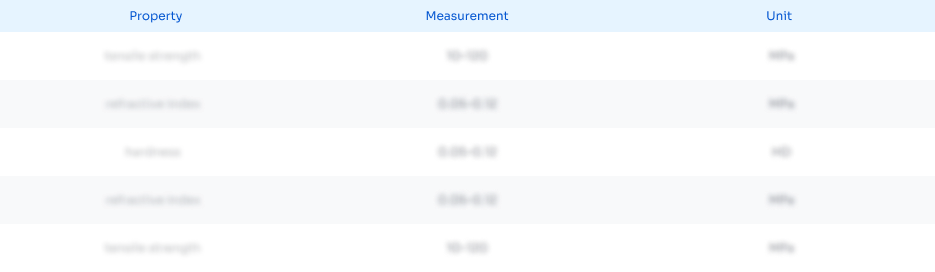
Abstract
Description
Claims
Application Information
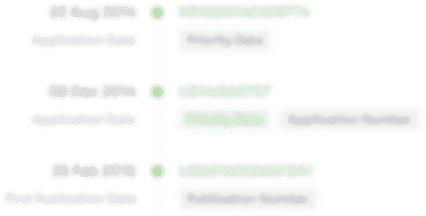
- R&D Engineer
- R&D Manager
- IP Professional
- Industry Leading Data Capabilities
- Powerful AI technology
- Patent DNA Extraction
Browse by: Latest US Patents, China's latest patents, Technical Efficacy Thesaurus, Application Domain, Technology Topic.
© 2024 PatSnap. All rights reserved.Legal|Privacy policy|Modern Slavery Act Transparency Statement|Sitemap