Grouping method of students' aerobic capacity driven by big data
A big data-driven, student-driven technology, applied in informatics, medical informatics, health index calculation, etc., can solve the problems of large amount of data, clustering algorithms that cannot perform iterative grouping operations, and cannot be loaded at the same time at one time. The effect of accuracy
- Summary
- Abstract
- Description
- Claims
- Application Information
AI Technical Summary
Problems solved by technology
Method used
Image
Examples
Embodiment Construction
[0046] The big data-driven student physique grouping method of the present invention will be further described in detail below in conjunction with the accompanying drawings.
[0047] The present invention solves the problem of grouping and clustering of students' aerobic capacity data. Among them, each student's aerobic capacity data is a numerical multivariate time series type, and the length of each aerobic capacity data is non-equal length. The representation of a piece of aerobic capacity data is shown in Table 1.
[0048] Table 1 A representation table of aerobic capacity data
[0049]
[0050] In the existing method, it is impossible to accurately compare the similarity of non-equal-length matrix data directly. The present invention proposes a two-stage clustering algorithm to solve the clustering problem of this type of data. The first stage adopts the HMM-based student's Oxygen capacity model generation algorithm, the second stage adopts hierarchical clustering alg...
PUM
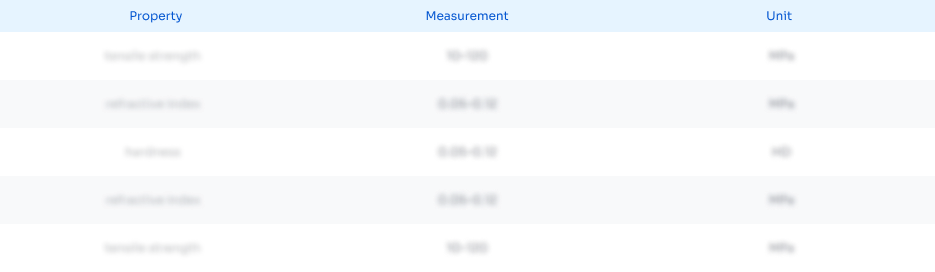
Abstract
Description
Claims
Application Information
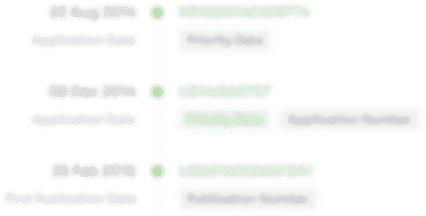
- R&D
- Intellectual Property
- Life Sciences
- Materials
- Tech Scout
- Unparalleled Data Quality
- Higher Quality Content
- 60% Fewer Hallucinations
Browse by: Latest US Patents, China's latest patents, Technical Efficacy Thesaurus, Application Domain, Technology Topic, Popular Technical Reports.
© 2025 PatSnap. All rights reserved.Legal|Privacy policy|Modern Slavery Act Transparency Statement|Sitemap|About US| Contact US: help@patsnap.com