Method for compressing deep neural network
A technology of neural network and compression method, which is applied in the direction of neural learning method, biological neural network model, neural architecture, etc., and can solve problems such as mode deviation
- Summary
- Abstract
- Description
- Claims
- Application Information
AI Technical Summary
Problems solved by technology
Method used
Image
Examples
Embodiment 1
[0070] Figure 8 A compression method suitable for an LSTM neural network according to the first embodiment of the present application is shown, wherein the compression of the neural network is realized through multiple iterative operations. Each iterative operation specifically includes three steps: sensitivity analysis, pruning, and retraining. Each step is described in detail below.
[0071] Step 8100, sensitivity test (Sensitivity analysis)
[0072] In this step, for example, sensitivity analysis is performed on all matrices in the LSTM network to determine the initial density (or initial compression ratio) of different matrices.
[0073] Figure 9 The specific steps of the sensitivity test are shown.
[0074] Such as Figure 9 As shown, in step 8110, for example, each matrix in the LSTM network is tried to be compressed according to different densities (the selected densities are, for example, 0.1, 0.2, ..., 0.9, for the specific compression method of matrices, refer...
Embodiment 2
[0178] In Embodiment 1 above, a method of compressing a trained dense neural network based on a fixed-shaped mask matrix to obtain a sparse neural network is proposed.
[0179] In Embodiment 2, the applicant proposes a new neural network compression method. In each round of compression, the method uses a user-designed dynamic compression strategy to compress the neural network.
[0180] Specifically, the dynamic compression strategy includes: the current number of times of pruning for each pruning operation, the maximum number of times of pruning, and the density of the pruning. These three parameters are used to determine the ratio of the weights that need to be pruned this time ( That is, the compression rate of this pruning operation), and pruning is performed based on this.
[0181] Therefore, in the neural network compression process according to the method of Embodiment 2, the strength of each pruning is a function of the number of pruning times (also can be understood a...
example 21
[0198] Example 2.1: Neural Network with Constant Density
[0199] In this example, the target density of each pruning operation is kept constant during one round of compression of the neural network. Accordingly, the compression function is:
[0200] f D (t)=D final
[0201] That is, in this round of compression, the density of the neural network is always kept constant, but the weight size and distribution can be changed.
[0202] Figure 16 Shows the neural network density variation curve in Example 2.1.
[0203] Figure 17 shown with Figure 16 The corresponding neural network weight distribution changes during the corresponding compression process.
[0204] Figure 17 The left side of the graph shows the changes in the weight parameter distribution of each matrix in the neural network during each pruning operation, where the horizontal axis shows the 9 matrices in each LSTM layer, and the vertical axis shows the pruning The number of operations. Visible, corre...
PUM
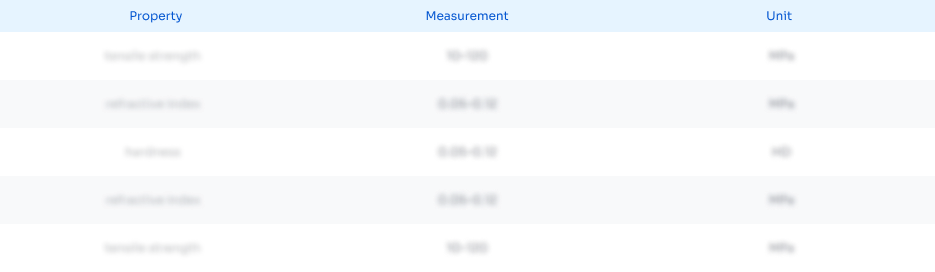
Abstract
Description
Claims
Application Information
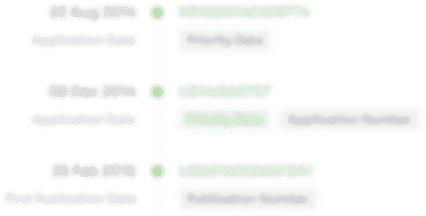
- R&D Engineer
- R&D Manager
- IP Professional
- Industry Leading Data Capabilities
- Powerful AI technology
- Patent DNA Extraction
Browse by: Latest US Patents, China's latest patents, Technical Efficacy Thesaurus, Application Domain, Technology Topic.
© 2024 PatSnap. All rights reserved.Legal|Privacy policy|Modern Slavery Act Transparency Statement|Sitemap