Image super-resolution method and system thereof
A super-resolution and low-resolution technology, applied in the field of image processing, can solve problems such as practical application limitations, increased computational complexity, and large training redundancy, and achieve the effect of reducing information redundancy, preventing gradient explosion, and enriching image information.
- Summary
- Abstract
- Description
- Claims
- Application Information
AI Technical Summary
Problems solved by technology
Method used
Image
Examples
Embodiment 1
[0062] figure 1 The main flowchart of the method is shown, including the following steps:
[0063] Step S110, preprocessing the input image.
[0064] Preprocessing involves upscaling the input image.
[0065] Including the following sub-steps:
[0066] Step S1101, input a low-resolution picture;
[0067] Step S1102, read the size of the high-resolution picture to be obtained;
[0068] Step S1103: Enlarge the low-resolution input picture based on quadratic cubic interpolation, so that the low-resolution input picture has the same size as the output picture.
[0069] Step S120, using the convolution network to realize the extraction and representation of the pixel block of the input picture.
[0070] Pre-construct a convolutional network of N (N is an integer greater than 1) layer, and use the convolutional network to realize the extraction and representation of the pixel block of the input image, including the following sub-steps:
[0071] Step S1201, using the first i (i...
Embodiment 2
[0080] The above describes how to use a convolutional network to convert a low-resolution image into a high-resolution image. In the current deep network training, since it is necessary to use the surrounding pixels to infer the central pixel, each time a convolutional layer of the convolutional network is added , the size of the feature map is reduced. For example: the size of the input image is (n+1)x(n+1), and when the receptive field of the network is nxn, the output image will be 1x1, where the receptive field refers to the output characteristics of each layer of the convolutional neural network The size of the area mapped by the pixels on the feature map on the original image.
[0081] This processing method prevents the pixels on the border of the image from using the surrounding pixels. The current processing method is to cut the border pixels. This is obviously not suitable when the axial area of the image is very large, because at this time, after cutting the su...
Embodiment 3
[0085] The image super-resolution method is described above through Embodiment 1 and Embodiment 2, and the low-resolution picture is converted into a high-resolution picture. The following describes the training method of the convolutional network used in the above-mentioned embodiment 1 in conjunction with the accompanying drawings, such as figure 2 shown, including the following steps:
[0086] Step S210, building a sample training database;
[0087] The sample training library includes multiple sets of training samples, and each set of training samples includes a low-resolution picture x and a high-resolution picture y corresponding to the low-resolution picture x.
[0088] Step S220, read a set of low-resolution pictures and their corresponding high-resolution pictures from the sample training database.
[0089] Step S230, calculating the loss function according to the low-resolution picture and the high-resolution picture; including the following sub-steps:
[0090] St...
PUM
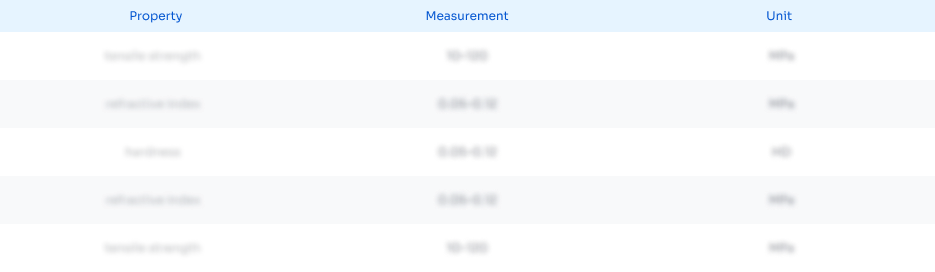
Abstract
Description
Claims
Application Information
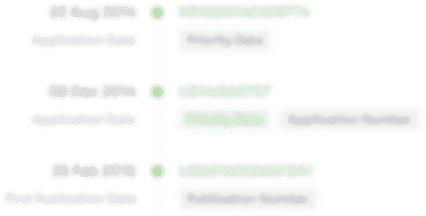
- R&D Engineer
- R&D Manager
- IP Professional
- Industry Leading Data Capabilities
- Powerful AI technology
- Patent DNA Extraction
Browse by: Latest US Patents, China's latest patents, Technical Efficacy Thesaurus, Application Domain, Technology Topic.
© 2024 PatSnap. All rights reserved.Legal|Privacy policy|Modern Slavery Act Transparency Statement|Sitemap