SOC-based data reuse convolutional neural network accelerator
A convolutional neural network and data multiplexing technology, applied in the field of convolutional neural networks for embedded devices, can solve problems such as low computing efficiency, time delay and power consumption waste, and achieve state machine simplification, area overhead and power consumption Small, the effect of improving computing efficiency
- Summary
- Abstract
- Description
- Claims
- Application Information
AI Technical Summary
Problems solved by technology
Method used
Image
Examples
Embodiment
[0094] The computational load of the convolutional neural network mainly includes image input, weight parameters and bias parameters of the convolutional neural network model itself. The feature of image input is that the two dimensions of the two-dimensional plane direction are relatively large, ranging from 1 to 107, and as the number of layers of the convolutional neural network deepens, the number of channels gradually increases, from 3 to 512; the weight parameter is generally Convolution kernel data, the two-dimensional plane direction dimensions are 7×7, 5×5, 3×3, 1×1, and the number of channels is 3 to 512; there is only one bias parameter for each channel, so each layer parameter is only 3 to 512 512. In view of these characteristics, the present invention stores different data separately, and adopts a block method, that is, group and store image input and weight parameters with large dimensions in the two-dimensional plane direction, and divide image storage and weig...
PUM
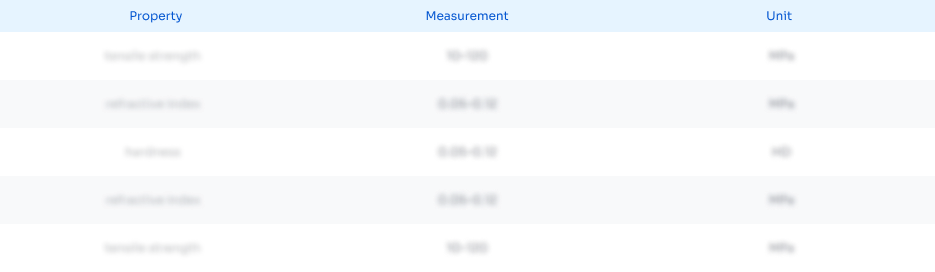
Abstract
Description
Claims
Application Information
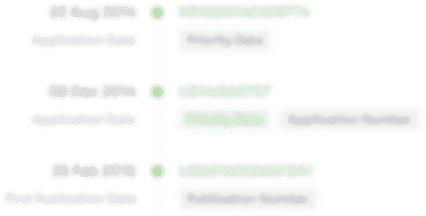
- R&D
- Intellectual Property
- Life Sciences
- Materials
- Tech Scout
- Unparalleled Data Quality
- Higher Quality Content
- 60% Fewer Hallucinations
Browse by: Latest US Patents, China's latest patents, Technical Efficacy Thesaurus, Application Domain, Technology Topic, Popular Technical Reports.
© 2025 PatSnap. All rights reserved.Legal|Privacy policy|Modern Slavery Act Transparency Statement|Sitemap|About US| Contact US: help@patsnap.com