Dynamic learning method and system for robot, robot and cloud server
A dynamic learning and robot technology, applied in the field of robot interaction, can solve the problems of inability to learn dynamically from people and the environment, and cannot establish deep artificial intelligence, so as to achieve the effect of improving user experience
- Summary
- Abstract
- Description
- Claims
- Application Information
AI Technical Summary
Problems solved by technology
Method used
Image
Examples
Embodiment 1
[0052] Please refer to figure 2 , this embodiment relates to a dynamic learning robot. In this embodiment, the rule base and annotation base are set on the robot terminal. The dynamic learning robot includes a training learning module 20 , a working module 30 , a task execution module 40 , a sending module 14 and an acquisition module 12 .
[0053] The training and learning module 20 includes a dynamic labeling module 22 , a rule updating module 26 and a labeling updating module 24 .
[0054] Wherein, the dynamic labeling module 22 dynamically labels the attribution-use relationship of objects and people in the three-dimensional environment, and stores it in the label library.
[0055] The dynamic labeling module 22 extracts the point cloud features of the 3D environment through machine vision, and obtains the appearance attributes (including but not limited to color, material), geometric model (object) of the object by identifying the point cloud and visual images. Shape),...
Embodiment 2
[0078] Please refer to image 3 , this embodiment also relates to a cloud server. In this embodiment, the rule base and annotation base are set on the cloud server to reduce the amount of data processing on the robot side and establish a unified artificial intelligence processing framework.
[0079] The cloud server includes a receiving module 102 , a sending module 104 , a storage module 120 , a rule library 122 , an annotation library 124 , a confirmation module 140 and an optimization module 130 .
[0080] The storage module 120 stores annotations from the robot that dynamically annotate the attribution-use relationship of objects and people in the three-dimensional environment, forming an annotation library 124 . The storage module also stores the robot's rule base 122 .
[0081] The receiving module 102 receives new rules established by the robot through interactive demonstration behaviors based on the rule base and annotation base. The receiving module 102 also receiv...
Embodiment 3
[0086] Please refer to figure 2, this embodiment relates to a dynamic learning system for a robot, and the dynamic learning system can be set separately on the robot side. For details, please refer to Embodiment 1. The dynamic learning system can also divide the work between the robot 10 and the cloud server 100 . When the cloud server 100 completes the establishment and updating of the annotation library and the rule library, please refer to Embodiment 2 for specific technical content.
[0087] In general, the robot dynamic learning system mainly includes a training learning module, a working module, a task execution module and an inquiry interaction module.
[0088] The training and learning module is used to perform the following steps: dynamically label the attribution and use relationship of objects and people in the three-dimensional environment to form a label library; obtain the rule library, and establish new rules and new labels based on the rule library and label ...
PUM
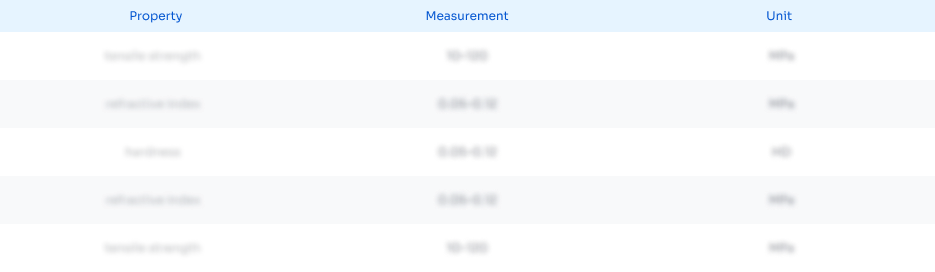
Abstract
Description
Claims
Application Information
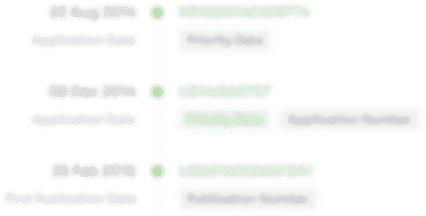
- R&D Engineer
- R&D Manager
- IP Professional
- Industry Leading Data Capabilities
- Powerful AI technology
- Patent DNA Extraction
Browse by: Latest US Patents, China's latest patents, Technical Efficacy Thesaurus, Application Domain, Technology Topic, Popular Technical Reports.
© 2024 PatSnap. All rights reserved.Legal|Privacy policy|Modern Slavery Act Transparency Statement|Sitemap|About US| Contact US: help@patsnap.com