Deep learning-based face recognition and face verification supervised learning method
A technology of supervised learning and face recognition, applied in the field of supervised learning, can solve the problems of increased computational complexity and inconvenient training process, and achieve the effect of stable face recognition, reduced intra-class distance and simple model
- Summary
- Abstract
- Description
- Claims
- Application Information
AI Technical Summary
Problems solved by technology
Method used
Image
Examples
Embodiment 1
[0052] In order to solve the problems revealed by the example model experiments, it is necessary to find a method that can reduce the intra-class distance and increase the inter-class distance of deep features. Studies have shown that increasing inter-class variables by extracting deep features from different people and reducing inter-class distance by clustering different features extracted from the same person are very important for face recognition and verification [11] . In this method, experiments are designed to explain how the parameter λ affects the outcome distribution in the proposed joint supervised learning, as Figure 4 demonstrates that different λ leads to different feature distributions. If the value of λ is appropriate, the distance between features of different categories increases, and the distance between features of the same category decreases, which significantly enhances the discriminative ability of deep features. Therefore, joint supervision benefits...
Embodiment 2
[0061] The scheme in embodiment 1 is further introduced below in conjunction with specific calculation formulas and examples, see the following description for details:
[0062] 201: Process large data sets, keep the number of characters in the data set unchanged, and reduce the number of pictures in the training data set to meet the protocol of small data sets;
[0063] The key feature points of faces and faces in all pictures are determined by the algorithm [13] detection. This method uses 5 key feature points of the face (two eyes, nose, two edges of the mouth) to do similarity transformation. For the training set, the image is simply discarded if the detection fails, but for the test set, the provided key features are used.
[0064] The image is cropped to a 112×96 RGB image. According to the convention of image preprocessing, each pixel in the RGB image (pixel value [0, 255]) is subtracted from 127.5, and then divided by 128 for normalization.
[0065] For the trainin...
Embodiment 3
[0102] The scheme in embodiment 1 and 2 is carried out feasibility verification below in conjunction with specific example, see the following description for details:
[0103] data set:
[0104] The LFW database is currently the most widely used face image database, such as Figure 5(a) and 5(b) shown. The database has a total of 13233 images, including 5749 different people. 1680 people had two or more images, and 4069 people had only one image. There are significant differences in pose, expression, and lighting between each image. Images are in JPEG format with a size of 250×250. The vast majority are color images, and a few are grayscale images. The database collects face pictures under natural conditions, and the purpose is to improve the accuracy of face recognition under natural conditions. There are 6 evaluation criteria for this dataset: Unsupervised; Image-restricted with no outside data; Unrestricted with no outside data; Unlabeled data Image-restricted with ...
PUM
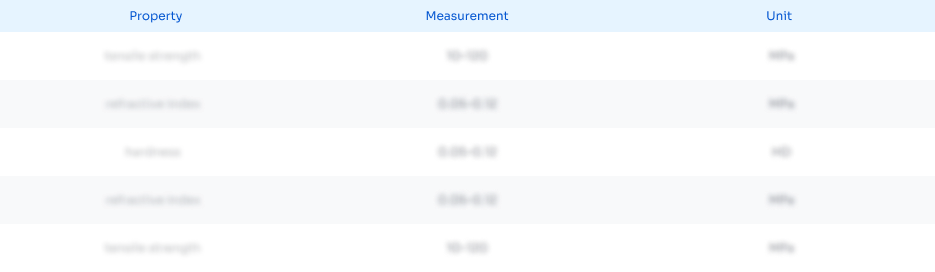
Abstract
Description
Claims
Application Information
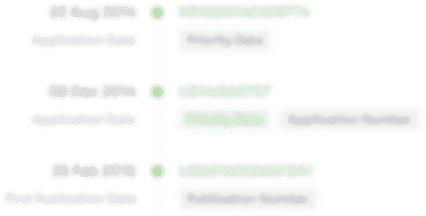
- R&D
- Intellectual Property
- Life Sciences
- Materials
- Tech Scout
- Unparalleled Data Quality
- Higher Quality Content
- 60% Fewer Hallucinations
Browse by: Latest US Patents, China's latest patents, Technical Efficacy Thesaurus, Application Domain, Technology Topic, Popular Technical Reports.
© 2025 PatSnap. All rights reserved.Legal|Privacy policy|Modern Slavery Act Transparency Statement|Sitemap|About US| Contact US: help@patsnap.com