Personnel reidentification method based on deep learning and distance metric learning
A distance measurement and deep learning technology, applied in the field of recognition, can solve the problems of poor generalization ability of similarity measurement model and poor pedestrian re-identification performance.
- Summary
- Abstract
- Description
- Claims
- Application Information
AI Technical Summary
Problems solved by technology
Method used
Image
Examples
Embodiment 1
[0099] Embodiment 1: The pedestrian re-identification method based on deep learning and distance metric learning in this embodiment
[0100] First of all, in view of the serious occlusion of video targets, a pedestrian target detection method based on convolutional neural network is used to process massive video data and detect pedestrian targets in the video. Secondly, the unsupervised RBM network is used to encode the initial features of the pedestrian target in a bottom-up manner to obtain a visual dictionary with both sparsity and selected rows; then, the initial visual dictionary is supervised and fine-tuned by error back propagation to obtain A new image expression method for video images, that is, image deep learning representation vectors; finally, the distance metric learning method of feature grouping and feature value optimization is used to obtain a metric space closer to the real semantics, and a linear SVM classifier is used to classify pedestrians target identif...
Embodiment 2
[0102] Embodiment 2: Due to the poor quality of the monitoring video, the uncontrollable environment, the wide range of shooting angles of view and the partial occlusion between pedestrians, it is difficult for the traditional target detection method to achieve good results in this open environment. This embodiment adopts a method based on The pedestrian target detection method of the convolutional neural network is mainly divided into the model training stage and the target detection stage. The specific process and results are as follows: figure 1 As shown, the specific process can be described as:
[0103] (1) In the model training stage, the focus of work is the selection and preprocessing of samples, as well as experimenting with CNN network parameters to select the optimal parameter combination. First, increase the diversity of samples by selecting samples from different angles and appearance colors, and then readjust these samples to a uniform resolution, and then enhanc...
Embodiment 3
[0105] Embodiment three: see figure 2 , image 3 , Figure 4 The pedestrian re-identification method based on the deep learning coding model of the present embodiment adopts the following steps to generate a visual dictionary with both sparseness and selectivity:
[0106] Figure 4 Among them, the visual dictionary represented by (a) is both sparse and selective, the visual dictionary represented by (b) is only selective, and the visual dictionary represented by (c) is only sparse.
[0107] First, extract the SIFT features of the training image library; extract the SIFT features; secondly, combine the spatial information of the SIFT features, use the adjacent SIFT features as the input of the RBM, train the RBM through the CD fast algorithm, and obtain the hidden layer features; then the adjacent hidden layer The features are used as the input of the next layer of RBM to get the output dictionary. Among them, ω 1 and ω 2 is the connection weight of RBM. RBM has an obvio...
PUM
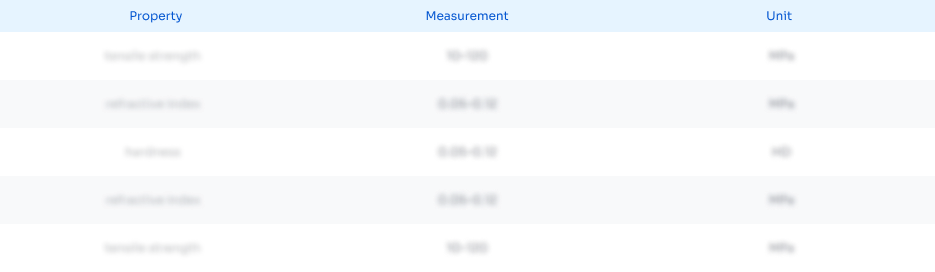
Abstract
Description
Claims
Application Information
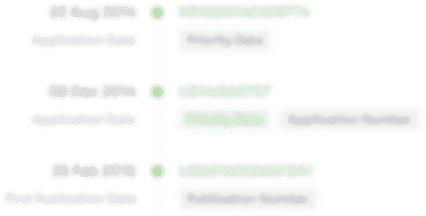
- Generate Ideas
- Intellectual Property
- Life Sciences
- Materials
- Tech Scout
- Unparalleled Data Quality
- Higher Quality Content
- 60% Fewer Hallucinations
Browse by: Latest US Patents, China's latest patents, Technical Efficacy Thesaurus, Application Domain, Technology Topic, Popular Technical Reports.
© 2025 PatSnap. All rights reserved.Legal|Privacy policy|Modern Slavery Act Transparency Statement|Sitemap|About US| Contact US: help@patsnap.com