Convolutional neural network method and support vector machine method-based image identification method for imaging department
A technology of convolutional neural network and support vector machine, which is applied in the field of image processing in medical imaging, can solve the problems that doctors do not have good recognition performance, the segmentation results and convergence are different, and the recognition ability is low, so as to achieve the ability to distinguish Strong, easy-to-implement, robustness-enhancing effects
- Summary
- Abstract
- Description
- Claims
- Application Information
AI Technical Summary
Problems solved by technology
Method used
Image
Examples
Embodiment Construction
[0012] Below in conjunction with accompanying drawing and embodiment the present invention will be further described:
[0013] Such as figure 1 Shown, a kind of imaging department image recognition method based on convolution neural network method and support vector machine method, it comprises the following steps:
[0014] Gather the original medical image as a sample, and use a weighted grayscale algorithm to grayscale the original medical image to obtain a grayscale image; use histogram equalization to process the grayscale image to obtain a grayscale image after equalization, preferably, The gray histogram is fitted with a high-order spline function, and the fitted curve has obvious valley points and peak points; the gray histogram after fitting is divided into valley value intervals, and the Obtain a smooth and derivable fitting curve on the basis of strip function fitting, and obtain the extreme point of the smooth and derivable fitting curve, and screen out the valley ...
PUM
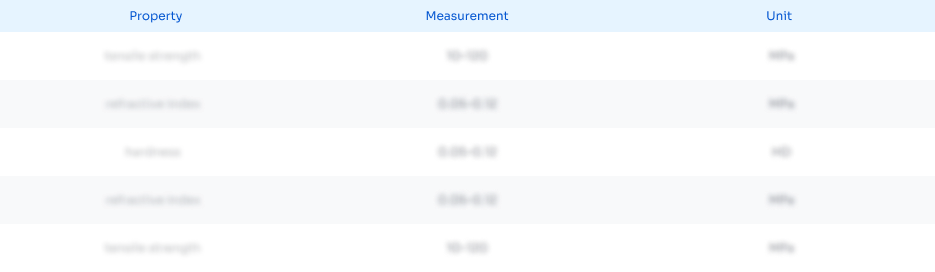
Abstract
Description
Claims
Application Information
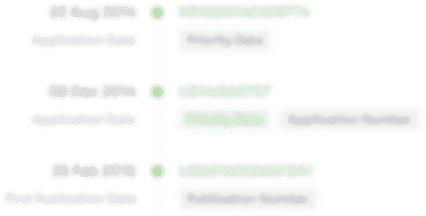
- R&D Engineer
- R&D Manager
- IP Professional
- Industry Leading Data Capabilities
- Powerful AI technology
- Patent DNA Extraction
Browse by: Latest US Patents, China's latest patents, Technical Efficacy Thesaurus, Application Domain, Technology Topic, Popular Technical Reports.
© 2024 PatSnap. All rights reserved.Legal|Privacy policy|Modern Slavery Act Transparency Statement|Sitemap|About US| Contact US: help@patsnap.com