Regional convolutional neural network-based efficient gesture detection and recognition method
A technology of convolutional neural network and gesture detection, which is applied in the field of efficient gesture detection and recognition based on regional convolutional neural network, can solve the problems of insufficient precision, limited input image size, and low accuracy, so as to improve the speed and accuracy of recognition rate effect
- Summary
- Abstract
- Description
- Claims
- Application Information
AI Technical Summary
Problems solved by technology
Method used
Image
Examples
Embodiment Construction
[0026] Below in conjunction with specific embodiment, further illustrate the present invention. It should be understood that these examples are only used to illustrate the present invention and are not intended to limit the scope of the present invention. In addition, it should be understood that after reading the teachings of the present invention, those skilled in the art can make various changes or modifications to the present invention, and these equivalent forms also fall within the scope defined by the appended claims of the present application.
[0027] Embodiments of the present invention relate to a static sign language real-time recognition method based on an improved single multi-target detector, such as figure 1 As shown, it includes the following steps: preprocessing the sample image of Chinese gesture letters; constructing and strengthening the gesture image data set, which is divided into training set and test set; using the Faster R-CNN network based on regiona...
PUM
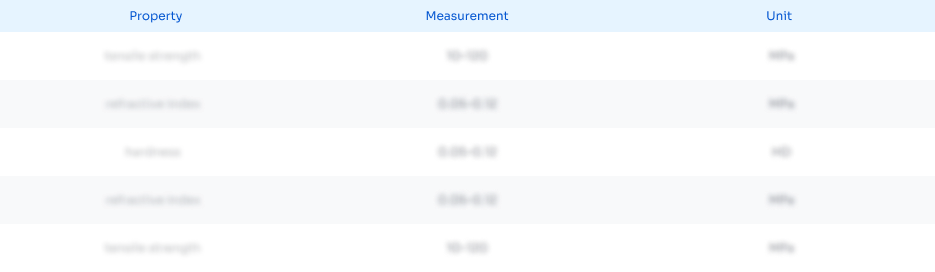
Abstract
Description
Claims
Application Information
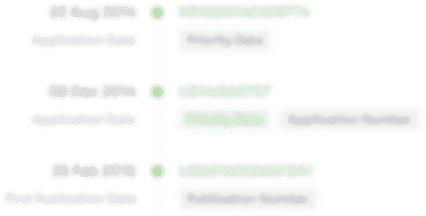
- R&D
- Intellectual Property
- Life Sciences
- Materials
- Tech Scout
- Unparalleled Data Quality
- Higher Quality Content
- 60% Fewer Hallucinations
Browse by: Latest US Patents, China's latest patents, Technical Efficacy Thesaurus, Application Domain, Technology Topic, Popular Technical Reports.
© 2025 PatSnap. All rights reserved.Legal|Privacy policy|Modern Slavery Act Transparency Statement|Sitemap|About US| Contact US: help@patsnap.com