Diabetic retinopathy classification method by using super lightweight SqueezeNet network
A diabetic retina and ultra-lightweight technology, applied in the field of deep learning, can solve problems such as the inability to make full use of medical image information, the accuracy cannot be improved, and the accuracy is limited, so as to improve the accuracy and reliability of classification and reduce the number of models The effect of the parameter
- Summary
- Abstract
- Description
- Claims
- Application Information
AI Technical Summary
Problems solved by technology
Method used
Image
Examples
Embodiment
[0036] Embodiment: a kind of diabetic retinopathy classification grading method (as figure 1 The flow chart of the method disclosed in the present invention), the core of the classification and grading method is: preparing a large number of SLO fundus photos for each type of diabetic retinopathy and performing preprocessing and data amplification; Convolutional neural network; based on a large number of ophthalmoscope photos, the deep convolutional neural network is trained, so that the final output value of the deep convolutional neural network conforms to the grading result of the ophthalmoscope photo; thus, the trained deep convolutional neural network can be used to automatically Perform disease classification.
[0037] The diabetic retinopathy classification and grading method using the ultra-lightweight SqueezeNet network includes the following steps:
[0038]1) Prepare a photo library, which contains several ophthalmoscope photos including diagnostic markers, and each ...
example 2,3,3.0,; pic 8(c) example 3
[0050] Figure 8 is an example of the four images and scores judged after normalization to probability. Figure 8(a) is example 1, the real label is class 0, the predicted probability of class 0 is 0.993, and the judgment is correct; Figure 8(b) is example 2, the real label is class 3, the predicted probability of class 3 is .0, and the judgment is correct ; Figure 8(c) is example 3, the real label is class 2, the predicted probability of class 1 is 0.562, and the judgment is wrong; Figure 8(d) is example 4, the real label is class 1, the predicted probability of class 1 is 0.613, and the judgment is correct.
[0051] Introduce the functional definitions of several main structures of the ultra-lightweight SqueezeNet network structure used in the present invention: In functional analysis, convolution, convolution or convolution are generated by two functions f and g to generate a third function A mathematical operator that characterizes the area of the overlapping part of the f...
PUM
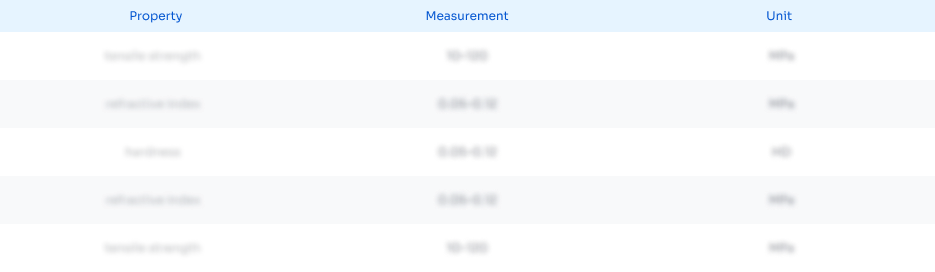
Abstract
Description
Claims
Application Information
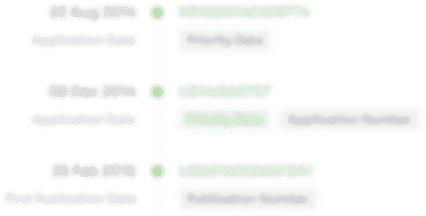
- R&D
- Intellectual Property
- Life Sciences
- Materials
- Tech Scout
- Unparalleled Data Quality
- Higher Quality Content
- 60% Fewer Hallucinations
Browse by: Latest US Patents, China's latest patents, Technical Efficacy Thesaurus, Application Domain, Technology Topic, Popular Technical Reports.
© 2025 PatSnap. All rights reserved.Legal|Privacy policy|Modern Slavery Act Transparency Statement|Sitemap|About US| Contact US: help@patsnap.com