Fault diagnosis method based on shafting rotation speed large fluctuation of automatic encoder
An autoencoder and fault diagnosis technology, which is applied in the testing of machines/structural components, instruments, mechanical bearings, etc., can solve problems that affect the final fault diagnosis effect and accuracy, model repetition training, etc., and achieve high accuracy, Improve accuracy and robustness
- Summary
- Abstract
- Description
- Claims
- Application Information
AI Technical Summary
Problems solved by technology
Method used
Image
Examples
Embodiment Construction
[0020] A method for diagnosing faults based on an automatic encoder for large fluctuations in the shafting speed of the present invention comprises the following steps:
[0021] Step 1. Sample data preprocessing: perform fast Fourier transform on the samples of speed 1 and speed 2 and normalize the amplitude;
[0022] Step 2. Pre-train the autoencoder: pre-train the autoencoder with the rev 1 signal;
[0023] Step 3. Train the transfer learning model based on the autoencoder: add the MMD penalty item to the autoencoder algorithm to automatically encode the transfer learning algorithm, and use the training samples of two rotation speeds for training;
[0024] Step 4. Train the Softmax-based migration learning classifier: Train the Softmax feature classifier with the MMD penalty item to classify the extracted features, so that fault diagnosis can be realized for the fault signal with unknown speed 2.
[0025] The present invention will be described in further detail below in co...
PUM
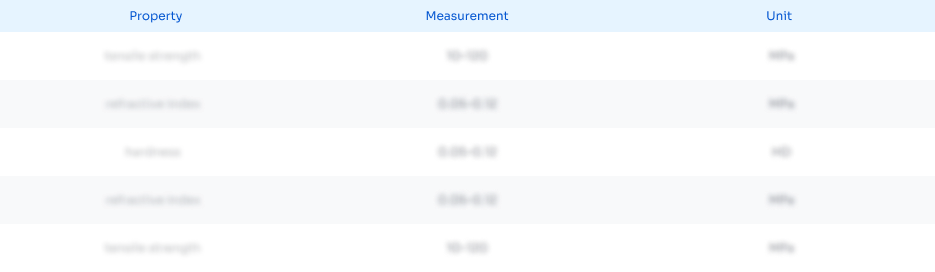
Abstract
Description
Claims
Application Information
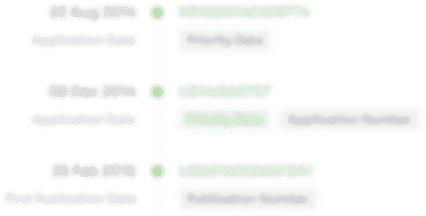
- R&D
- Intellectual Property
- Life Sciences
- Materials
- Tech Scout
- Unparalleled Data Quality
- Higher Quality Content
- 60% Fewer Hallucinations
Browse by: Latest US Patents, China's latest patents, Technical Efficacy Thesaurus, Application Domain, Technology Topic, Popular Technical Reports.
© 2025 PatSnap. All rights reserved.Legal|Privacy policy|Modern Slavery Act Transparency Statement|Sitemap|About US| Contact US: help@patsnap.com