Method for automatically segmenting liver tumors in abdominal CT sequence images
A technology for sequential images and liver tumors, applied in the field of image processing, can solve problems such as complex structures, blurred borders of liver tumors, and low contrast
- Summary
- Abstract
- Description
- Claims
- Application Information
AI Technical Summary
Problems solved by technology
Method used
Image
Examples
Embodiment 1
[0037] In order to obtain the liver region in the abdominal CT sequence images, the automatic abdominal CT sequence liver segmentation method published in the document "A hierarchical local region-based sparse shape composition for liver segmentation in CT scans" (pattern recognition, pp.88-106, 2016.) was used. The original CT sequence images are preprocessed to obtain the liver region in the sequence. figure 1 (a)-(d) are 4 original images randomly selected from a certain CT sequence, figure 2 (a)-(d) are the liver segmentation results obtained by using the method of this embodiment, that is, the liver region mask.
Embodiment 2
[0039] A liver area enhancement method for abdominal CT sequence images, the specific implementation steps are as follows:
[0040] (1) Using Embodiment 1 to obtain the liver region in the abdominal CT sequence image f;
[0041] (2) In order to obtain the gray distribution range of the liver region, a Gaussian function is used to fit the gray histogram of the entire liver region in the sequence:
[0042]
[0043] Among them, c is the peak value of the Gaussian distribution, and μ and σ are the center and standard deviation of the Gaussian distribution, respectively. image 3 for right figure 1 The Gaussian fitting result of the gray histogram of the liver area in the sequence image shown, it can be seen that the gray level of the liver can better conform to the Gaussian distribution. According to the probability theory of Gaussian distribution, the gray ranges of [μ-σ,μ+σ], [μ-2σ,μ+2σ] and [μ-3σ,μ+3σ] occupy 68%, 95%, 99% of the pixels. Considering the noise and tumor t...
Embodiment 3
[0050] Using Example 2 to obtain liver enhancement results, combined with image boundary information, construct a graph cut energy function for multi-target segmentation:
[0051]
[0052] Among them, α is a normal number from 0 to 1, P represents all pixel sets in the abdominal CT sequence image f, N p Represents the neighborhood pixel set of pixel p, R(f p ) and B(f p , f q ) are the grayscale and boundary penalty items, and are obtained by the following formulas:
[0053]
[0054]
[0055] in
[0056]
[0057]
[0058] f p and f q respectively represent the pixel points p and q in the image f, I p and I q Represents the gray value of pixels p and q, d(p,q) represents the Euclidean distance between pixels p and q, T P Indicates the total number of pixels in the pixel set P of the image f, mask is the liver mask obtained in Example 1, the pixels belonging to the liver area are marked as 1, and the pixels belonging to the background are marked as 0, that...
PUM
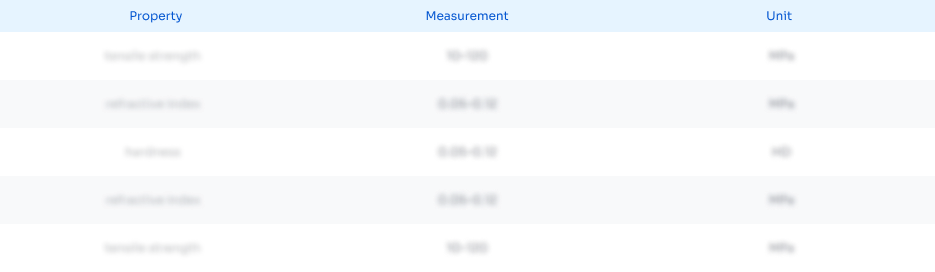
Abstract
Description
Claims
Application Information
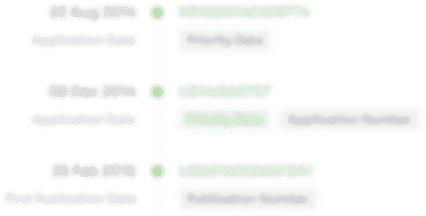
- R&D
- Intellectual Property
- Life Sciences
- Materials
- Tech Scout
- Unparalleled Data Quality
- Higher Quality Content
- 60% Fewer Hallucinations
Browse by: Latest US Patents, China's latest patents, Technical Efficacy Thesaurus, Application Domain, Technology Topic, Popular Technical Reports.
© 2025 PatSnap. All rights reserved.Legal|Privacy policy|Modern Slavery Act Transparency Statement|Sitemap|About US| Contact US: help@patsnap.com