Privacy-protecting multi-party deep learning computation proxy method under cloud environment
A deep learning and privacy protection technology, applied in the field of cloud computing, can solve the problems of low model accuracy, differential privacy privacy leakage, and difficulty in wide application of multi-party deep learning.
- Summary
- Abstract
- Description
- Claims
- Application Information
AI Technical Summary
Problems solved by technology
Method used
Image
Examples
Embodiment Construction
[0044] The invention belongs to the technical field of cloud computing, and discloses a deep learning method for multi-party privacy protection in a cloud environment. The deep learning of multi-party privacy protection in the cloud environment is realized, in which the data set in the model is distributed in the databases of multiple users. Using the novel multi-party deep learning method proposed in the present invention, users can obtain a unified deep learning model based on integrated data sets, and at the same time, privacy protection of each user data set can be realized, which solves the problem of privacy leakage in multi-party machines. At the same time, the present invention proposes a method for realizing the verifiability of the proxy calculation result by using the aggregated signature, so as to ensure the correctness of the result.
[0045] Aiming at the problems existing in the prior art, the present invention provides a privacy-protecting multi-party deep lear...
PUM
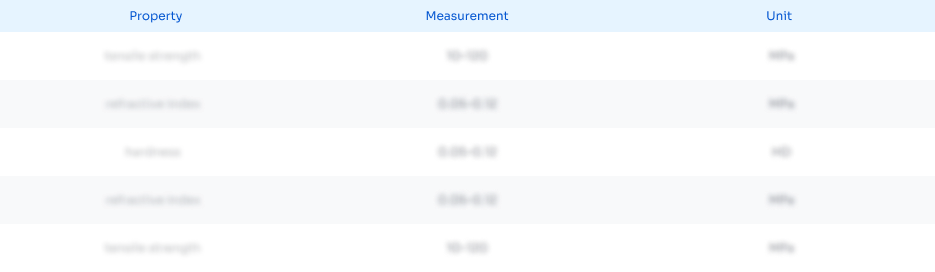
Abstract
Description
Claims
Application Information
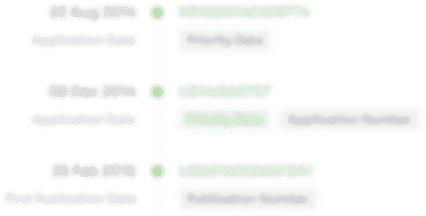
- R&D Engineer
- R&D Manager
- IP Professional
- Industry Leading Data Capabilities
- Powerful AI technology
- Patent DNA Extraction
Browse by: Latest US Patents, China's latest patents, Technical Efficacy Thesaurus, Application Domain, Technology Topic, Popular Technical Reports.
© 2024 PatSnap. All rights reserved.Legal|Privacy policy|Modern Slavery Act Transparency Statement|Sitemap|About US| Contact US: help@patsnap.com