Top-N movie recommendation method for performing weighted fusion on selected local models based on random anchor points
A technology of weighted fusion and local models, which is applied in electrical digital data processing, special data processing applications, instruments, etc., can solve problems such as over-fitting, achieve the effect of maintaining stability and solving over-fitting
- Summary
- Abstract
- Description
- Claims
- Application Information
AI Technical Summary
Problems solved by technology
Method used
Image
Examples
Embodiment Construction
[0062] refer to figure 1The general flow chart of the technical solution, the present invention has seven stages in total, namely: data preprocessing stage, user feature vector calculation stage, movie feature vector calculation stage, local training matrix construction stage, local recommendation model training stage, local recommendation model weighted fusion stage and the model validation stage. The data preprocessing stage is to clean the data set, remove some inactive users and unpopular movies, construct a corpus for LDA topic model training and a user movie implicit feedback training matrix for sparse linear model training; user feature vector calculation In the stage, the feature vector of users at the semantic level is calculated by using the LDA topic model; in the movie feature vector calculation stage, the feature vector of the movie in the semantic level is reconstructed by using the GBDT gradient boosting decision tree; in the local training matrix construction s...
PUM
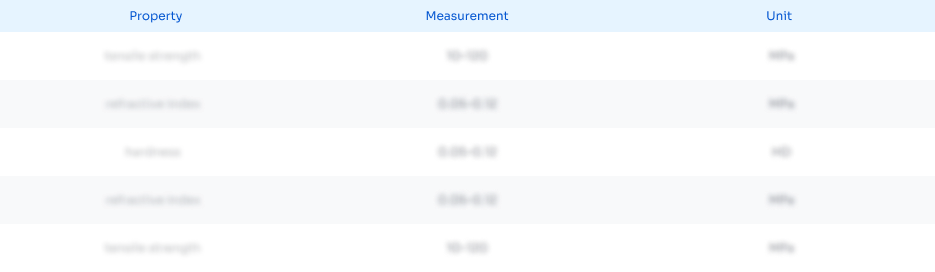
Abstract
Description
Claims
Application Information
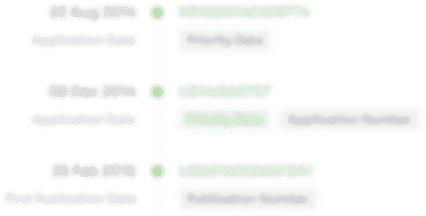
- R&D Engineer
- R&D Manager
- IP Professional
- Industry Leading Data Capabilities
- Powerful AI technology
- Patent DNA Extraction
Browse by: Latest US Patents, China's latest patents, Technical Efficacy Thesaurus, Application Domain, Technology Topic, Popular Technical Reports.
© 2024 PatSnap. All rights reserved.Legal|Privacy policy|Modern Slavery Act Transparency Statement|Sitemap|About US| Contact US: help@patsnap.com