Method for constructing virtual case library of cancer pathological images and multi-scale cancer detection system based on convolutional neural network
A convolutional neural network, pathological image technology, applied in biological neural network models, image enhancement, neural architecture, etc., can solve problems such as low work efficiency, difficult diagnosis in the medical field, and inability to meet the needs of image reading, and improve time efficiency. , the effect of reducing computing resources
- Summary
- Abstract
- Description
- Claims
- Application Information
AI Technical Summary
Problems solved by technology
Method used
Image
Examples
Embodiment 1
[0035] Example 1 Taking the multi-scale detection method of breast cancer lymphatic metastasis as an example
[0036]The specific meaning of the breast cancer lymphatic metastasis task is to automatically detect whole-section pathological images of lymphatic metastasis stained by hematoxylin and eosin (H&E). This task is of high clinical interest, but requires significant time-consuming manual reading by pathologists. Therefore, an effective computer vision automatic detection scheme can reduce the workload of pathologists while improving the objectivity of diagnosis.
[0037] Such as figure 1 and figure 2 The flow chart shown is a multi-scale detection system for lymphatic metastasis of breast cancer based on convolutional neural network. Based on the method of convolutional neural network, it detects the cancer mass area on the pathological full scan slice, including the following modules:
[0038] Pathological slice image preprocessing module: on a high scale, convert ...
Embodiment 2
[0092] Similarly, the convolutional neural network-based multi-scale cancer detection system described in Example 1 can also be used for the detection of gastric cancer, and the breast cancer tissue is replaced by gastric cancer tissue, and the detection method is to use the above-mentioned detection system, Take the following steps:
[0093] Step (1), tissue area extraction: obtain gastric cancer patient tissue, preprocess the pathological full-scan slice image, convert the full-scan slice image from RGB color space to HSL color space, use the high-scale whole slice image, and use the S-channel The Otsu algorithm was used to perform threshold segmentation on it to obtain tissue regions. ;
[0094] Step (2), construction of virtual case database: use the deep convolutional generative confrontation network algorithm to train the generative model, and use the generative model to generate low-scale sliced images, then down-sample the generated images, generate a series of slic...
Embodiment 3
[0102]Similarly, the convolutional neural network-based multi-scale cancer detection system described in Example 1 can also be used for the detection of rectal cancer, and breast cancer tissue is replaced by rectal cancer tissue, and the detection method is to use the above-mentioned detection system, the following steps are taken:
[0103] Step (1), tissue area extraction: obtain rectal cancer patient tissue, preprocess the pathological full-scan slice image, convert the full-scan slice image from RGB color space to HSL color space, use the high-scale whole slice image, and use S Channels were thresholded using the Otsu algorithm to obtain tissue regions. ;
[0104] Step (2), construction of virtual case database: use the deep convolutional generative confrontation network algorithm to train the generative model, and use the generative model to generate low-scale sliced images, then down-sample the generated images, generate a series of sliced images and compare them to ...
PUM
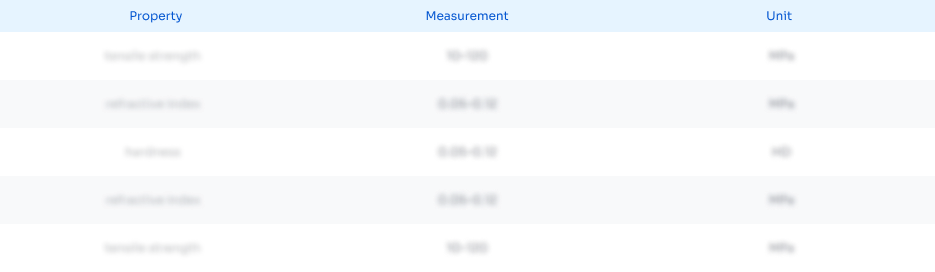
Abstract
Description
Claims
Application Information
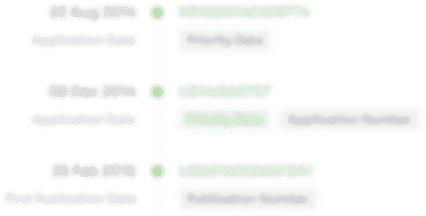
- R&D
- Intellectual Property
- Life Sciences
- Materials
- Tech Scout
- Unparalleled Data Quality
- Higher Quality Content
- 60% Fewer Hallucinations
Browse by: Latest US Patents, China's latest patents, Technical Efficacy Thesaurus, Application Domain, Technology Topic, Popular Technical Reports.
© 2025 PatSnap. All rights reserved.Legal|Privacy policy|Modern Slavery Act Transparency Statement|Sitemap|About US| Contact US: help@patsnap.com