Video action recognition method and device
A technology of action recognition and video recognition, applied in the fields of computer vision and machine learning, can solve the problems of difficult to obtain data training network, lack of quality control of video, and difficult to expand to large-scale data sets, etc., to achieve good and effective recognition Effect
- Summary
- Abstract
- Description
- Claims
- Application Information
AI Technical Summary
Problems solved by technology
Method used
Image
Examples
Embodiment 1
[0048] figure 1 is a schematic flow chart of the video action recognition method provided in Embodiment 1 of the present invention, as figure 1 as shown,
[0049] The video action recognition method that the embodiment of the present invention provides, comprises the following steps:
[0050] 101. Extract the spatio-temporal features of the video.
[0051] Specifically, the time-series segmentation network is used to extract the spatio-temporal features of the video. The process includes:
[0052] The spatial convolutional network and temporal convolutional network included in the time series segmentation network extract static image features and moving optical flow features respectively, and generate corresponding feature vectors. Temporal SegmentNetwork (TSN) is used to extract the spatio-temporal features of each segment (reference [2]. Limin Wang, Yuanjun Xiong, Zhe Wang, YuQiao, DahuaLin, Xiaoou Tang, and Luc Van Gool. 2016. Temporal segmentnetworks : Towards good pra...
Embodiment 2
[0070] Figure 5 It is a flow chart of the video action recognition method provided by Embodiment 2 of the present invention, such as figure 2 As shown, the video action recognition method provided by the embodiment of the present invention includes the following steps:
[0071] 201. Perform video preprocessing on the video and the video to be recognized, where the video preprocessing includes video segment segmentation and key frame extraction.
[0072] Specifically, the video for training and recognition and the video to be recognized will be required, and the pictures of RGB static frames and the optical flow pictures of motion will be extracted.
[0073] It should be noted that the process implemented in step 201 can be implemented in other ways besides the ways described in the above steps, and the embodiment of the present invention does not limit the specific way.
[0074] 202. Extract the spatio-temporal features of the video through a temporal segmentation network....
Embodiment 3
[0088] Figure 6 is a schematic structural diagram of a video action recognition device provided by an embodiment of the present invention, as shown in Figure 6 As shown, the video action recognition device provided by the embodiment of the present invention mainly includes an extraction module 31 , a training module 32 and a recognition module 33 .
[0089] Specifically, the extraction module 31 is used to extract the spatio-temporal features of the video, specifically extracting static image features and moving optical flow features through the spatial convolutional network and temporal convolutional network included in the temporal segmentation network, and generating corresponding features vector. The time node for the extraction module to extract the spatio-temporal features of the video is to extract the spatio-temporal features of the video to be identified, or, before using the target dense expansion network model to identify the video to be identified, the extractio...
PUM
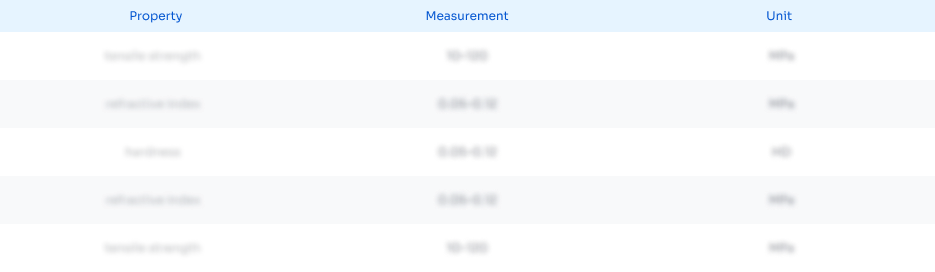
Abstract
Description
Claims
Application Information
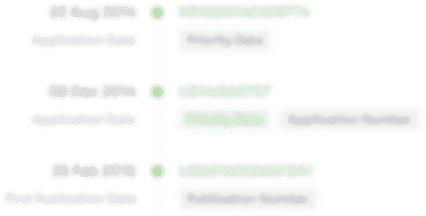
- R&D Engineer
- R&D Manager
- IP Professional
- Industry Leading Data Capabilities
- Powerful AI technology
- Patent DNA Extraction
Browse by: Latest US Patents, China's latest patents, Technical Efficacy Thesaurus, Application Domain, Technology Topic, Popular Technical Reports.
© 2024 PatSnap. All rights reserved.Legal|Privacy policy|Modern Slavery Act Transparency Statement|Sitemap|About US| Contact US: help@patsnap.com