Unmanned vehicle semantic map modeling and application constructing method based on perception and location monitoring
A technology of semantic maps and modeling methods, applied in directions such as road network navigators, can solve the problems of not using the correlation of map elements, not making good use of the correlation of map elements, and lack of semantic information, so as to improve the efficiency of correlation search. Effect
- Summary
- Abstract
- Description
- Claims
- Application Information
AI Technical Summary
Problems solved by technology
Method used
Image
Examples
specific Embodiment 1
[0041] Such as figure 1 , figure 2 As shown, this embodiment provides a semantic map modeling method, including the conceptual structure of the semantic map, semantic relations, and a method for generating a semantic map by instantiating a real map.
[0042] Such as image 3 As shown, the semantic ontology is divided into two modules: entities and attributes:
[0043] (1) Entities include self-vehicle, road network entity and obstacle entity, respectively representing the self-vehicle (unmanned vehicle) entity, road network element entity and obstacle entity.
[0044] (11) The self-vehicle refers to the unmanned vehicle itself, which can be expanded to different types of unmanned vehicles according to the needs.
[0045] (12) Road network entities include area entities and point entities, representing area type entities and point type entities respectively.
[0046] (121) Regional entities include overall road sections, connection points, boundaries, road separation strip...
specific Embodiment 2
[0060] Such as Image 6 As shown, the method of static map data instantiation and real-time obstacle instantiation to generate a semantic map, the steps are as follows:
[0061] Step 1: Obtain detailed data information of the real driving environment through perception systems such as lidar, camera, GPS, and satellite photos, and instantiate the detailed map data into static road network entities according to the conceptual structure of the map;
[0062] Step 2: Obtain real-time obstacle pose information through sensors such as lidar, camera, and GPS, and instantiate the obstacle information into an obstacle map entity;
[0063] Step 3: Establish the semantic relationship between the entities in the static map obtained in Step 1 and Step 2 and the obstacle map, and finally obtain the semantic map for unmanned vehicles.
specific Embodiment 3
[0064] Such as Figure 7 As shown, it is a modeling example diagram of a real map, which includes a crossroad, a U-turn, multiple road sections and other map elements. The key elements are marked with arrows, and the ground signs and roadside signs are only Take one as an illustration.
[0065] Firstly, the detailed map data is obtained; then, the detailed map data is divided into different types of map elements according to the conceptual structure of the semantic map and instantiated into static road network entities according to the aforementioned conceptual structure.
[0066] As shown in the figure, the horizontal and vertical roads represent two overall road segment entities. The intersection entity is connection point 002, and the U-turn entity is connection point 001. Each road segment is connected to other road segments through connection points. The middle of the road The dotted arrow represents the connection constraint entity, which is associated with the connecti...
PUM
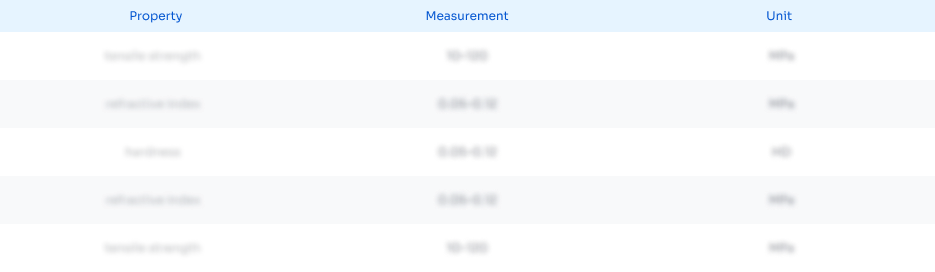
Abstract
Description
Claims
Application Information
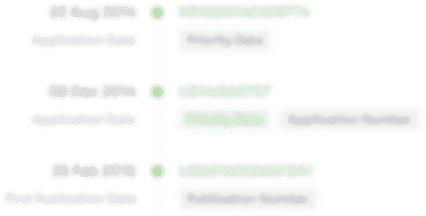
- R&D Engineer
- R&D Manager
- IP Professional
- Industry Leading Data Capabilities
- Powerful AI technology
- Patent DNA Extraction
Browse by: Latest US Patents, China's latest patents, Technical Efficacy Thesaurus, Application Domain, Technology Topic.
© 2024 PatSnap. All rights reserved.Legal|Privacy policy|Modern Slavery Act Transparency Statement|Sitemap