A weighted Gaussian model soft measurement modeling method with time delay estimation
A technology of Gaussian model and modeling method, applied in the direction of calculation, design optimization/simulation, special data processing application, etc., can solve the problems of poor model prediction accuracy, high computational complexity, unsatisfactory nonlinear process, etc. The effect of increasing output, improving product quality and reducing production costs
- Summary
- Abstract
- Description
- Claims
- Application Information
AI Technical Summary
Problems solved by technology
Method used
Image
Examples
Embodiment 1
[0071] This embodiment provides a weighted Gaussian model soft sensor modeling method with time delay estimation, the method comprising:
[0072] Step 1: Obtain historical sampling input and output data to form a historical training sample database; determine the maximum delay parameter T according to the process mechanism and experience max ;
[0073] Step 2: Normalize the training sample data in the historical training sample database, and determine the optimal delay parameter of each input variable through the sliding gray correlation analysis algorithm, and the optimal delay parameter is defined as λ 1 ,λ 2 ,,,λ m ; where m is the dimension of the training sample;
[0074] Step 3: Perform data reconstruction on the training sample data according to the optimal delay parameters determined by the sliding gray correlation analysis algorithm;
[0075] Step 4: When querying sample x q When it arrives, the online pair queries sample x q Create a weighted Gaussian model.
...
Embodiment 2
[0078] This embodiment provides a weighted Gaussian model soft sensor modeling method with time delay estimation, see figure 2 , this embodiment takes a common chemical process—the debutanizer process as an example; the experimental data comes from the debutanizer process, and the butane concentration at the bottom of the debutanizer is predicted.
[0079] Step 1: Obtain historical sampling input and output data to form a historical training database; determine the maximum delay parameter T according to the process mechanism and experience max .
[0080] Step 2: Normalize the training sample data, and determine the optimal delay parameter of each input variable through Moving gray correlation analysis (MGRA), which is defined as λ 1 ,λ 2 ,...,λ m ; where m is the dimension of the sample;
[0081] The sliding gray correlation degree algorithm is:
[0082] MGRA is used to select important input variables: by constructing the functional relationship between input and output...
PUM
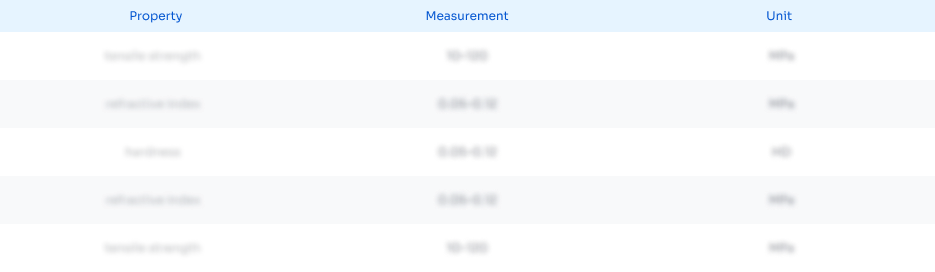
Abstract
Description
Claims
Application Information
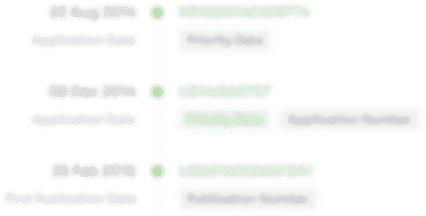
- R&D Engineer
- R&D Manager
- IP Professional
- Industry Leading Data Capabilities
- Powerful AI technology
- Patent DNA Extraction
Browse by: Latest US Patents, China's latest patents, Technical Efficacy Thesaurus, Application Domain, Technology Topic, Popular Technical Reports.
© 2024 PatSnap. All rights reserved.Legal|Privacy policy|Modern Slavery Act Transparency Statement|Sitemap|About US| Contact US: help@patsnap.com