A fault identification method of analog circuit based on improved limit learning machine
A technology for simulating circuit faults and extreme learning machines, which can be used in analog circuit testing, neural learning methods, electronic circuit testing, etc., and can solve problems such as lack of modeling flexibility in diagnostic models.
- Summary
- Abstract
- Description
- Claims
- Application Information
AI Technical Summary
Problems solved by technology
Method used
Image
Examples
Embodiment
[0072] figure 1 It is a flow chart of the fault identification method for analog circuits based on the improved extreme learning machine of the present invention.
[0073] In this example, if figure 1 Shown, the present invention a kind of analog circuit fault identification method based on improved extreme learning machine, comprises the following steps:
[0074] S1. Collect voltage eigenvalues at different corner frequencies
[0075] Such as figure 2 As shown, analyze the corner frequency of the analog circuit, and collect the voltage eigenvalues of N groups of samples at different corner frequencies in the fault state and healthy state of the analog circuit, denoted as X={x 1 ,x 2 ,...,x i ,...,x N}, where the voltage eigenvalue x i ={x i,1, x i,2 ,...,x i,k ...,x i,T},x i,k Indicates the voltage value of the i-th sample collected at the k-th corner frequency, and T is the number of corner frequencies;
[0076] Construct the expected output vector Y={y for...
example
[0137] In order to illustrate the technical effects of the present invention, an analog circuit is taken as an example to verify the implementation of the present invention. Such as image 3 As shown, the circuit is composed of 4 second-order filters and an adder, and it is modeled and simulated using Pspice software. The tolerance of R1, R2, R3, R4, R5, R6, R7 and R8 is ±10%, the tolerance of C1, C2, C3, C4, C5, C6, C7 and C8 is ±5%, the gain of the amplifier is Av1 , the tolerance of Av2, Av3 and Av4 is ±1%, and the tolerance of R9, R10 and R11 is ±1%. Analysis shows that the circuit has 4 corner frequencies: 10Hz, 100Hz, 10kHz and 100kHz. The probability of a single fault in an analog circuit accounts for about 80%, so only the state monitoring and health management of a single fault is considered.
[0138] Set Av 1 ,Av 2 ,Av 3 and Av 4 In (1.1~1.5%)Xn, (1.6~2%)Xn, (2.1~2.5%)Xn, (2.6~3.0%)Xn, (3.1~3.5%)Xn, (3.6~4.0%)Xn, ( 4.1~4.5%)Xn, (4.6~5.0%)Xn, (5.1~5.5%)Xn, (5....
PUM
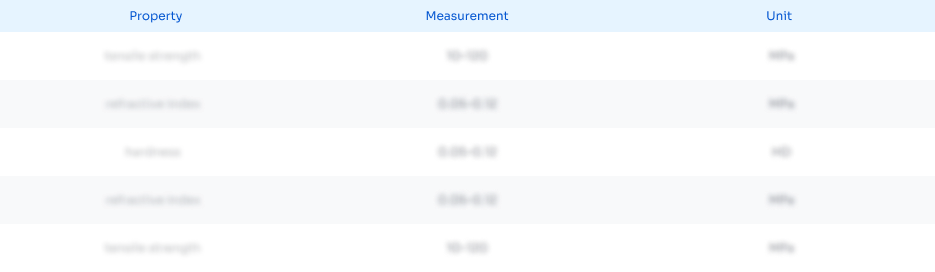
Abstract
Description
Claims
Application Information
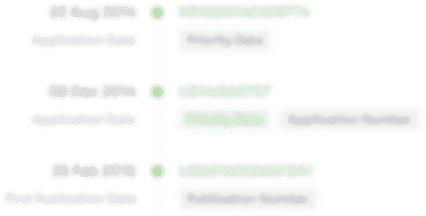
- R&D
- Intellectual Property
- Life Sciences
- Materials
- Tech Scout
- Unparalleled Data Quality
- Higher Quality Content
- 60% Fewer Hallucinations
Browse by: Latest US Patents, China's latest patents, Technical Efficacy Thesaurus, Application Domain, Technology Topic, Popular Technical Reports.
© 2025 PatSnap. All rights reserved.Legal|Privacy policy|Modern Slavery Act Transparency Statement|Sitemap|About US| Contact US: help@patsnap.com