Mechanical fault diagnosis method for unsupervised deep learning network
A deep learning network, mechanical failure technology, applied in neural learning methods, testing of mechanical components, biological neural network models, etc., can solve the difficulty of manual labeling information, the difficulty of obtaining label information of mechanical equipment, and the lack of data information sparseness of label information. and other problems to achieve the effect of enhancing the generalization ability
- Summary
- Abstract
- Description
- Claims
- Application Information
AI Technical Summary
Problems solved by technology
Method used
Image
Examples
Embodiment example 1
[0110] Implementation Case 1: In this section, in order to verify the performance of the proposed UDLN-based mechanical fault diagnosis model, various types of rolling bearing faults need to be simulated. The experiment is performed on an accelerated bearing life tester (ABLT-1A provided by Hangzhou Bearing Test Research Center). The main components of the test bench are: computer control system, test head seat, test head, lubrication system, transmission system, loading system, Test and data acquisition system. The designed tester has four bearings mounted on a shaft driven by an AC motor, and its transmission system is supported by rubber belts for connecting the AC motor and the shaft using two pulleys. At the same time, various faults of 6205 single-row deep groove ball bearings were simulated experimentally. In the experiment, wire cutting was used to simulate the faults of 6205 rolling bearings, including inner ring faults, composite faults of inner and outer rings, com...
PUM
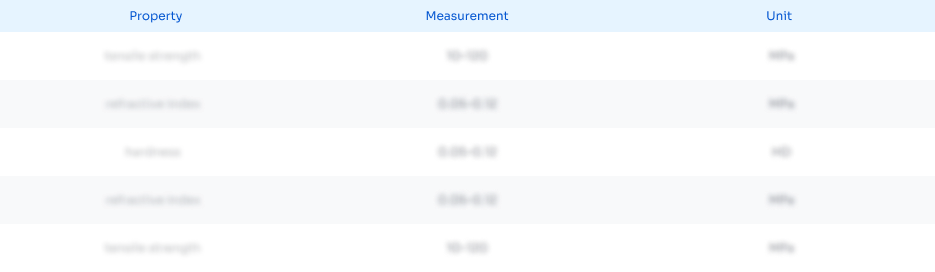
Abstract
Description
Claims
Application Information
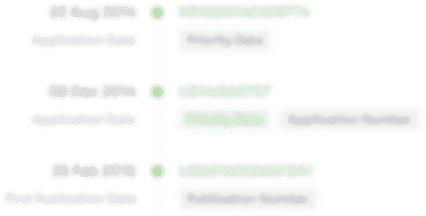
- R&D Engineer
- R&D Manager
- IP Professional
- Industry Leading Data Capabilities
- Powerful AI technology
- Patent DNA Extraction
Browse by: Latest US Patents, China's latest patents, Technical Efficacy Thesaurus, Application Domain, Technology Topic, Popular Technical Reports.
© 2024 PatSnap. All rights reserved.Legal|Privacy policy|Modern Slavery Act Transparency Statement|Sitemap|About US| Contact US: help@patsnap.com